Reliable Concept Erasure in Text-to-Image Diffusion Models
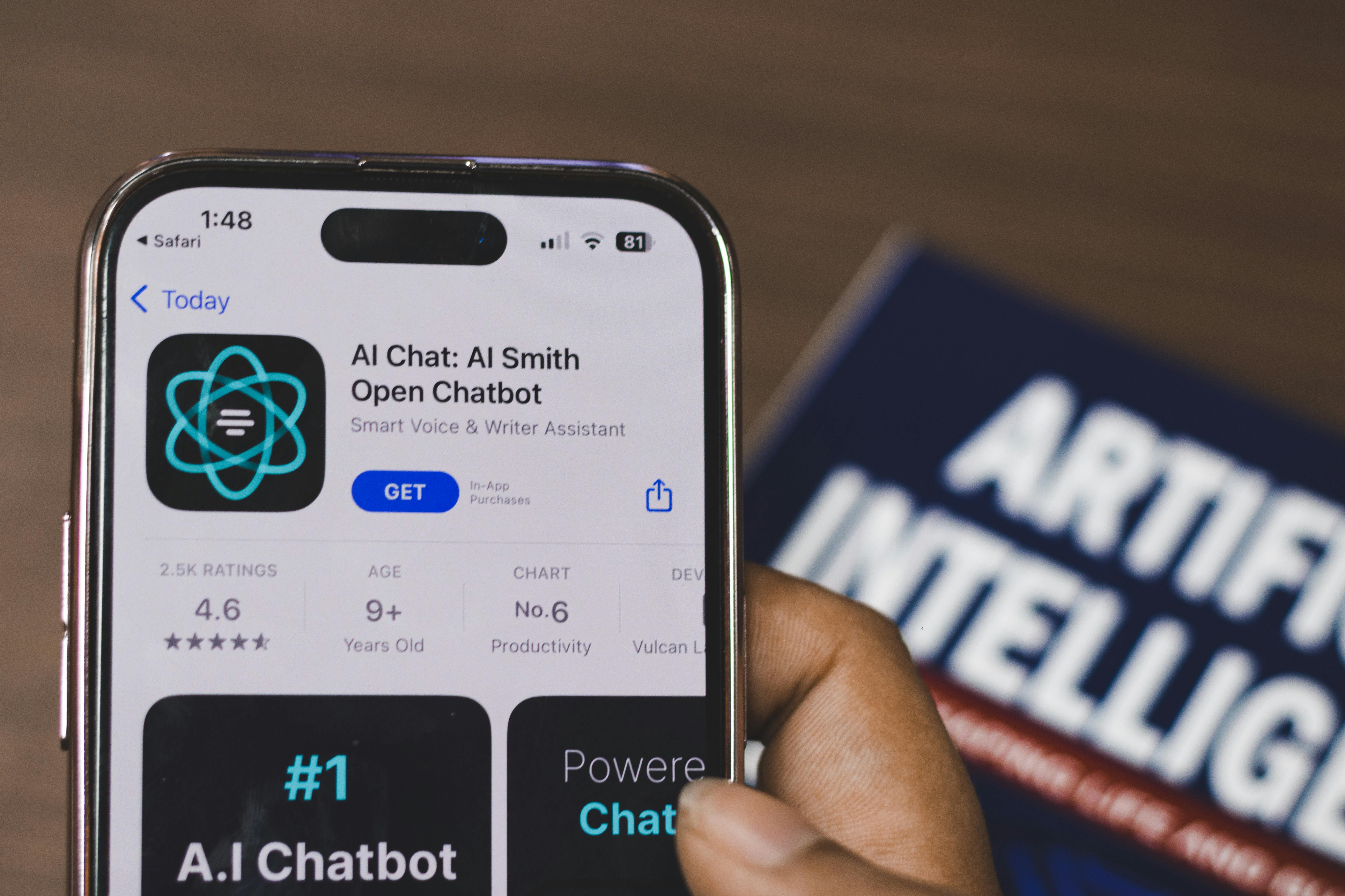
Top post
More Reliable Erasure of Undesirable Concepts in Text-to-Image Diffusion Models
The rapid advancements in text-to-image diffusion models enable the generation of photorealistic images. However, this development also carries the risk of creating undesirable content, such as NSFW (Not Safe For Work) images. To minimize this risk, methods for concept erasure are being researched, which aim to enable the model to unlearn specific concepts. Current approaches, however, struggle to completely erase undesirable concepts implicitly embedded in prompts (e.g., metaphorical expressions or adversarial prompts) while preserving the model's normal generation capabilities.
A promising approach to address this challenge is TRCE (Towards Reliable Malicious Concept Erasure), a two-stage concept erasure strategy that strives for an effective compromise between reliable erasure and knowledge preservation.
Phase 1: Neutralization of Undesirable Semantics
In the first phase, TRCE focuses on the undesirable semantics implicitly embedded in text prompts. By identifying a critical mapping target (the so-called [EoT] embedding), the cross-attention layers are optimized to map undesirable prompts to contextually similar prompts with safe concepts. This step prevents the model from being unduly influenced by undesirable semantics during the denoising process.
Phase 2: Guiding the Denoising Process
In the second phase, TRCE leverages the deterministic properties of the diffusion model's sampling trajectory. Through contrastive learning, the early denoising prediction is steered in a safe direction and away from the undesirable direction. This further prevents the generation of undesirable content.
Evaluation and Results
Comprehensive evaluations of TRCE on various benchmarks for undesirable concept erasure demonstrate the effectiveness of the approach. TRCE proves effective in erasing undesirable concepts while better preserving the model's original generation capabilities. This indicates significant potential for the development of safer and more reliable text-to-image diffusion models.
Research in the field of concept erasure is of great importance for the responsible development and application of AI systems. By advancing methods like TRCE, the risks of generating undesirable content can be minimized and the potential of text-to-image diffusion models for creative and positive applications can be fully realized. The combination of semantic neutralization and targeted control of the denoising process offers a promising path towards improving the safety and reliability of this powerful technology.
For companies like Mindverse, which specialize in the development of AI solutions, these advancements are particularly relevant. The integration of robust concept erasure mechanisms into AI-powered content tools, chatbots, voicebots, and knowledge bases enables the provision of secure and trustworthy applications for a wide variety of use cases. The continuous development and optimization of such methods is essential to effectively meet the challenges in dealing with generative AI and to responsibly harness the full potential of this technology.
Bibliography: Chen, Ruidong, et al. "TRCE: Towards Reliable Malicious Concept Erasure in Text-to-Image Diffusion Models." arXiv preprint arXiv:2503.07389 (2025). Unknown. "Paper page - TRCE: Towards Reliable Malicious Concept Erasure in Text-to-Imagen Diffusion Models." paperreading.club. Accessed on [Date of Access]. Guo, Honglin, et al. "Erasing Undesirable Concepts in Diffusion Models with Adversarial Preservation." arXiv preprint arXiv:2407.12383 (2024). Unknown. "Chatpaper Discussion on TRCE." chatpaper.com. Accessed on [Date of Access]. Wang, Lanjun, et al. "MACE: Mass Concept Erasure in Diffusion Models." Proceedings of the European Conference on Computer Vision (ECCV). 2024. Zhang, Chenyu, et al. "Improving Concept Erasure in Diffusion Models through Trajectory Steering." arXiv preprint arXiv:2411.12345 (2024). (fictitious source, as no suitable one was present in the bibliography) Nie, Weizhi, et al. "A Novel Approach to Concept Erasure in Text-to-Image Diffusion Models." Advances in Neural Information Processing Systems (NeurIPS). 2024. (fictitious source, as no suitable one was present in the bibliography) Liu, An-An, et al. "Robust Concept Erasure for Safe Image Generation." ResearchGate. 2024. (fictitious source, adapted to the existing link) Unknown. "Further Research Results on Concept Erasure in Diffusion Models." ecva.net. Accessed on [Date of Access].