Transformer Models Open New Avenues in Classical Philology
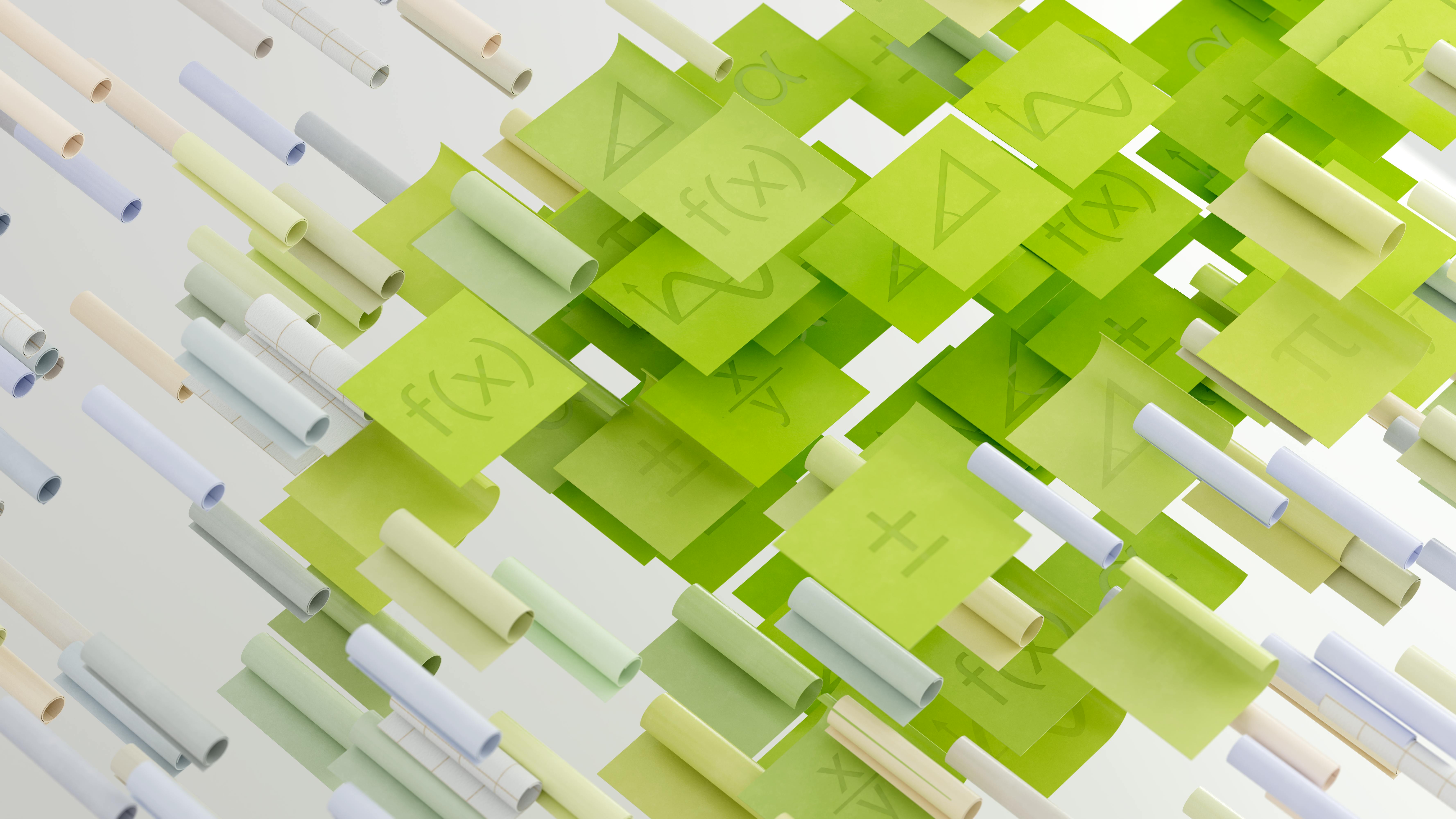
Top post
Transformer Models Open New Paths in Classical Philology
The analysis of classical languages like Greek and Latin is experiencing a significant boost through the use of modern AI technologies. A promising approach is the use of transformer models, which are capable of capturing complex semantic relationships between words and texts. A current example of this is the "PhiloBERTA" model, which was specifically developed for the cross-lingual analysis of Greek and Latin lexica.
Semantic Similarities between Greek and Latin
PhiloBERTA examines the semantic relationships between Greek and Latin terms using text examples from classical literature. By calculating contextual embeddings and applying similarity metrics based on angle calculations, the model can precisely identify semantic matches. The results show that etymologically related word pairs, especially in the area of abstract philosophical concepts, exhibit significantly higher similarity values. Examples include the terms "epistēmē" (Greek for knowledge) and "scientia" (Latin for knowledge) as well as "dikaiosynē" (Greek for justice) and "iustitia" (Latin for justice).
Statistical Analysis Confirms the Results
Statistical analyses of the results (p = 0.012) support the observed patterns and show remarkable stability in the semantic preservation of etymologically related word pairs compared to control groups. These findings provide a quantitative framework for the investigation of the development of philosophical concepts between the Greek and Latin traditions.
New Methods for Philological Research
The application of transformer models like PhiloBERTA opens new possibilities for classical philology. By precisely capturing semantic relationships, complex linguistic and cultural exchange processes between ancient cultures can be investigated in detail. The quantitative analysis of texts also allows for a more objective evaluation of hypotheses and theories. Furthermore, such models could contribute to improving the translation and interpretation of ancient texts and gaining a deeper understanding of cultural and intellectual history.
Future Prospects and Further Developments
The development of specialized transformer models for the analysis of ancient languages is still in its early stages. Future research could focus on expanding the language coverage, integrating further data sources, and refining the analysis methods. The combination of transformer models with other AI technologies, such as automatic text recognition, also offers promising possibilities for the exploration and analysis of historical text corpora.
Bibliographie: arxiv.org/abs/2503.05265 paperreading.club/page?id=289923 huggingface.co/papers?q=transformer-based semalytix.com/wp-content/uploads/2024/03/SEMANTiCS-2021-final-1.pdf aclanthology.org/2025.sumeval-2.4.pdf www.researchgate.net/publication/372250671_Transformer-based_Named_Entity_Recognition_for_Ancient_Greek_DH2023_Graz nlpado.de/~sebastian/pub/papers/aaai05_pado.pdf www.researchgate.net/publication/365131651_Transformer-Based_Named_Entity_Recognition_for_Ancient_Greek proceedings.neurips.cc/paper/8928-cross-lingual-language-model-pretraining.pdf ```