Scaling Laws for Native Multimodal Models Challenge Late-Fusion Dominance
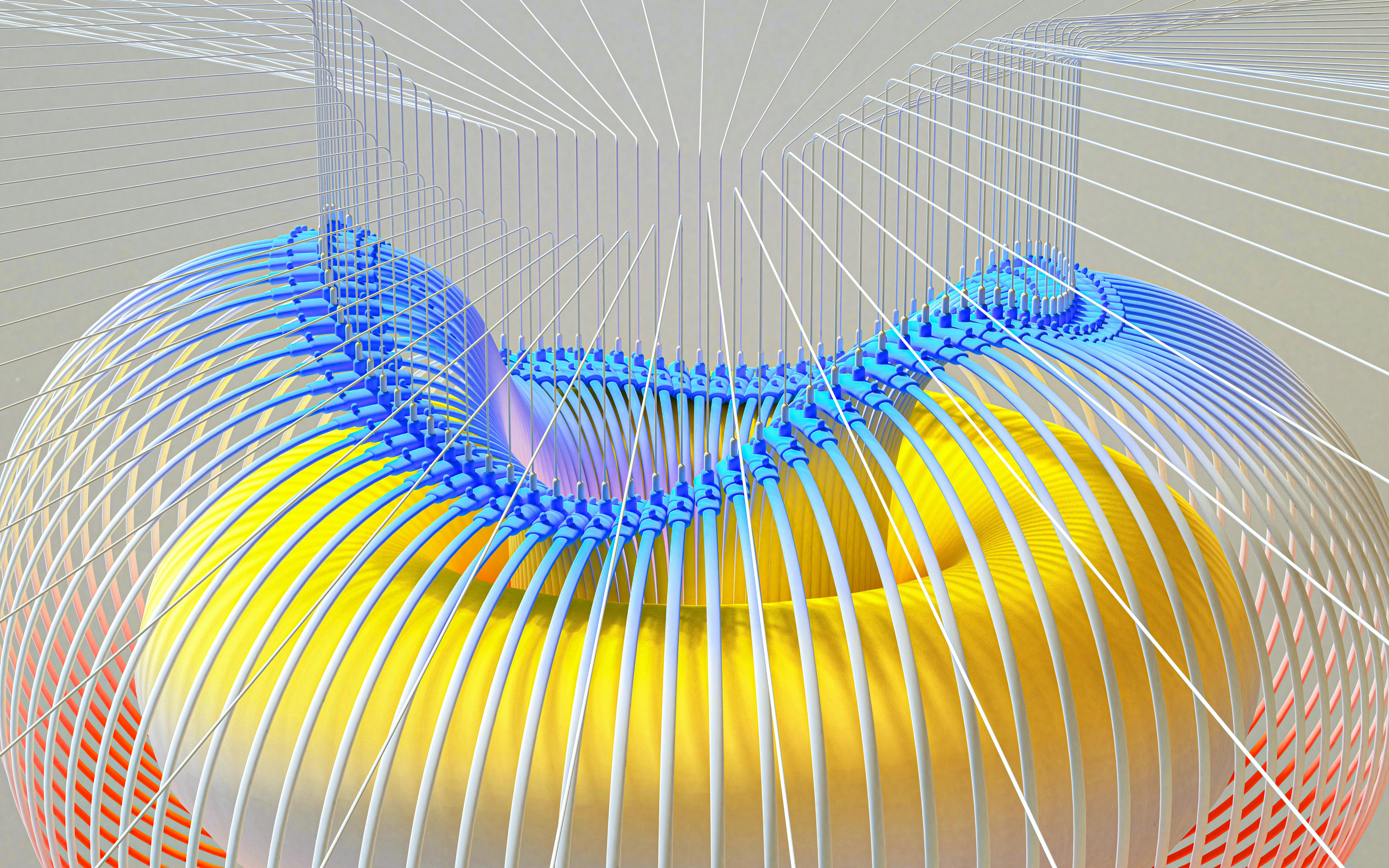
Top post
Scaling Laws for Native Multimodal Models: A New Look at Architecture and Efficiency
The development of AI models that are multimodal, meaning they can perceive and process the world through different sensory channels like text and images, is a central research area. The current trend is to combine individually pre-trained components, such as image encoders and large language models (LLMs), and then further train them multimodally. These so-called late-fusion architectures show remarkable sample efficiency, but their fundamental superiority over other architectures has remained unclear.
A new study now investigates the architecture of native multimodal models (NMMs), which are trained from scratch with all modalities. In a comprehensive analysis of 457 trained models with different architectures and training data, scaling laws were examined. The results challenge the perceived dominance of late-fusion architectures.
Early-Fusion: Efficient and Powerful
The study shows that early-fusion architectures, which do without separate image encoders, achieve comparable, sometimes even better performance with fewer parameters. Moreover, they are more efficient to train and easier to implement. In early fusion, the different modalities are processed together from the beginning, in contrast to late fusion, where the modalities are first processed separately and only combined later. This advantage of early-fusion models with fewer parameters is particularly relevant with regard to the resources and costs required for training large AI models.
Mixture of Experts (MoE): Modality-Specific Weighting
Building on the promising results of early-fusion architectures, the study also investigated the use of Mixture of Experts (MoE). This technique allows models to learn modality-specific weightings, leading to a significant performance increase. MoE models consist of several expert networks, each specialized in certain aspects of the data. A so-called gating network decides which expert is best suited for the respective input. In the context of multimodal models, experts can be trained for processing text, images, or other modalities.
Implications for the Future of Multimodal AI
The results of this study have far-reaching implications for the development of future multimodal AI systems. The finding that early-fusion architectures, especially in combination with MoE, represent a powerful and efficient alternative to the established late-fusion approaches opens new avenues for research and development. The simplified architecture and reduced training effort could accelerate and democratize the development and deployment of multimodal AI models in various application areas. Further research is necessary to fully exploit the potential of these architectures and to understand the scaling laws for even larger and more complex multimodal models.
Bibliography Shukor, M., Fini, E., Turrisi da Costa, V. G., Cord, M., Susskind, J., & El-Nouby, A. (2025). Scaling Law Hypothesis for Multimodal Model. *arXiv preprint arXiv:2504.07951*. Aghajanyan, A., Dziri, N., Glaese, A., Goyal, N., Heffernan, D., Jain, A., ... & Zettlemoyer, L. (2023). Scaling language models: Methods, analysis & insights from training gopher. *arXiv preprint arXiv:2203.15556*. Bugliarello, E. (2023). *Scaling Laws and Emergent Abilities of Large Language Models* (Doctoral dissertation, University of Copenhagen).