RayFlow Accelerates Image Generation with Instance-Aware Diffusion
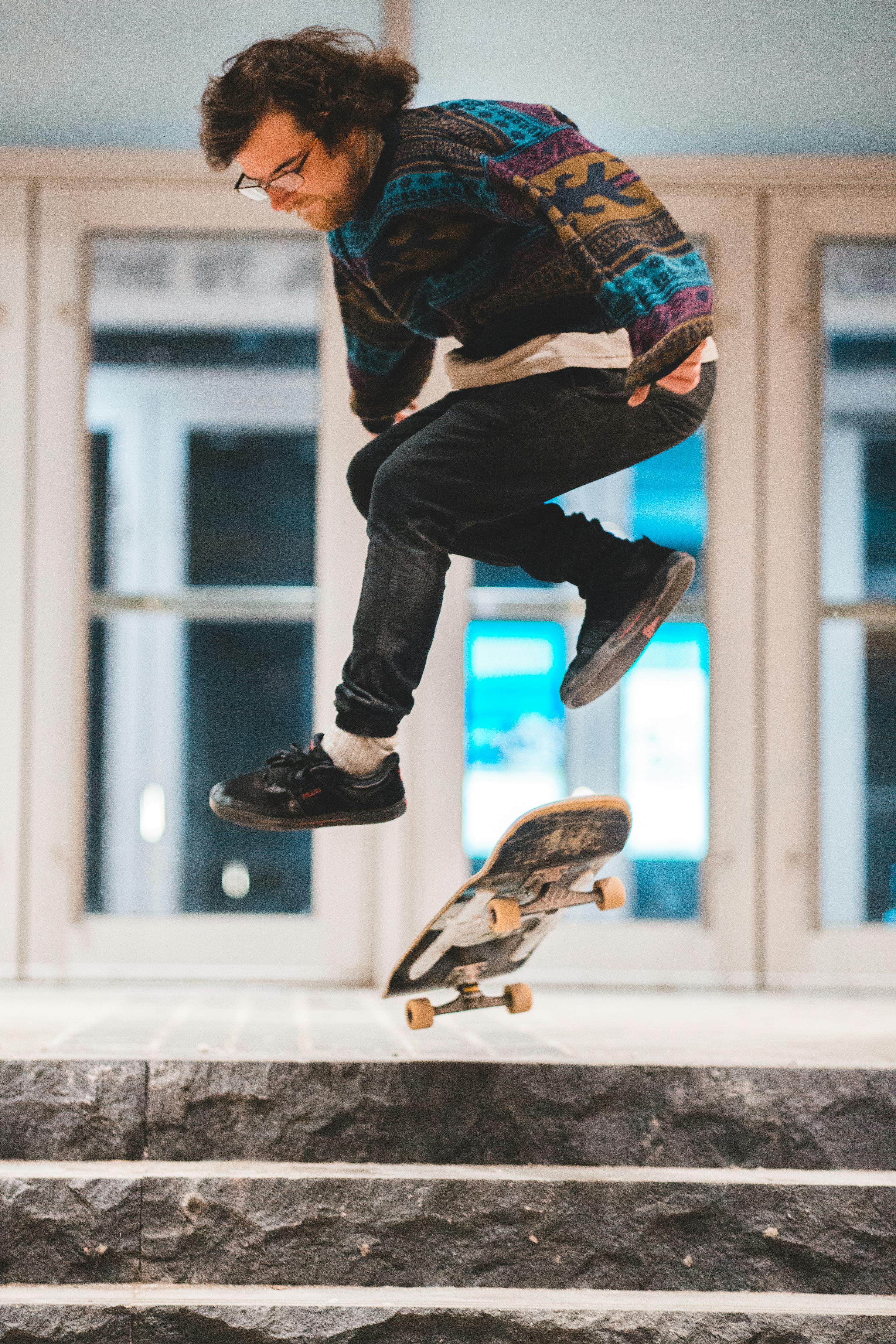
Top post
Faster and More Efficient Image Generation with RayFlow: A New Approach for Diffusion Models
Diffusion models have made impressive progress in image generation in recent years. From creating photorealistic images to artistic design, they open up diverse possibilities. A significant disadvantage of these models, however, is their comparatively slow generation speed. Calculating the many steps required to generate an image from random noise requires considerable computing power and time. Various acceleration methods have already been developed to reduce the number of steps. However, this often comes at the expense of image quality, the controllability of the process, or leads to increased complexity in training the models.
A promising solution to this problem presents itself in the form of RayFlow, a novel framework for diffusion models. RayFlow takes an innovative approach by guiding each sample along an individual path to an instance-specific target distribution. In contrast to conventional methods, which generate all samples along the same path, RayFlow enables targeted and efficient computation. This minimizes the number of necessary steps without compromising the diversity and stability of the generated images.
The core of RayFlow lies in the adaptive control of the "trajectories," i.e., the paths that the samples traverse during the generation process. These trajectories are dynamically adapted to the respective instance to find the fastest possible route to the target distribution. This approach allows for a significant acceleration of generation without affecting the quality of the results.
In addition to the adaptive control of trajectories, RayFlow integrates another innovative technique called "Time Sampler." This is an importance sampling method that increases training efficiency by focusing on the most important time steps. By selectively choosing the most relevant time steps, the computational effort is reduced without compromising the quality of the training.
Extensive experiments demonstrate the performance of RayFlow. Compared to existing acceleration techniques, RayFlow generates high-quality images at increased speed and simultaneously offers more control over the generation process. The improved training efficiency through the Time Sampler further contributes to the attractiveness of the framework.
For companies like Mindverse, which specialize in AI-powered content creation, RayFlow offers the potential to significantly accelerate and optimize image generation. Integration into all-in-one content tools could lead to a significant improvement in workflows and user experience. The development of customized solutions, such as chatbots, voicebots, AI search engines, and knowledge systems, could also benefit from the advantages of RayFlow.
Bibliography: - Huiyang Shao, Xin Xia, Yuhong Yang, Yuxi Ren, Xing Wang, Xuefeng Xiao. "RayFlow: Instance-Aware Diffusion Acceleration via Adaptive Flown Trajectories." arXiv preprint arXiv:2503.07699 (2025). - Proceedings of the IEEE/CVF Conference on Computer Vision and Pattern Recognition (CVPR). 2025. - Proceedings of the IEEE/CVF Conference on Computer Vision and Pattern Recognition (CVPR). Current. - Proceedings of the European Conference on Computer Vision (ECCV). 2024. - Proceedings of the International Conference on Medical Image Computing and Computer Assisted Intervention (MICCAI). 2023.