SANA-Sprint: Ultra-Fast Image Generation with One-Step Diffusion
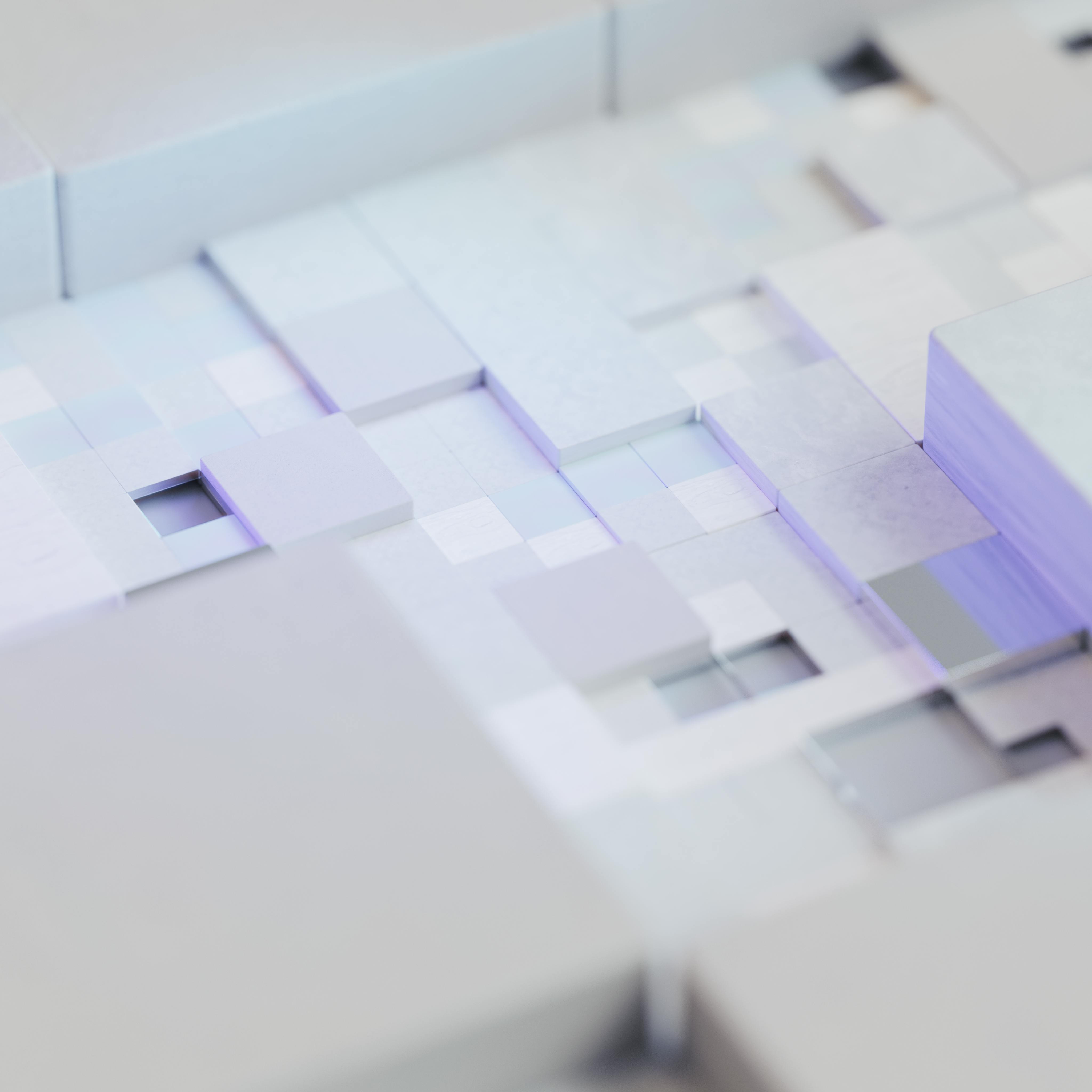
Top post
SANA-Sprint: A New Approach for Ultra-Fast Image Generation
The development of text-to-image (T2I) models has made rapid progress in recent years. A key focus is on improving speed and efficiency without sacrificing the quality of the generated images. SANA-Sprint presents itself as a promising approach that addresses precisely this challenge.
The Core Innovations of SANA-Sprint
SANA-Sprint is based on a pre-trained foundation model and uses hybrid distillation to reduce the number of inference steps from typically 20 to 1-4. Three central innovations distinguish SANA-Sprint:
First, SANA-Sprint uses a training-free method that transforms a pre-trained flow-matching model for Continuous-Time Consistency Distillation (sCM). This eliminates the need for time-consuming training from scratch and significantly increases training efficiency. The hybrid distillation strategy combines sCM with latent adversarial distillation (LADD). While sCM ensures consistency with the teacher model, LADD improves the quality of the images generated in a single step.
Second, SANA-Sprint is a unified, step-adaptive model that generates high-quality images in just 1-4 steps. This eliminates the need for step-specific training, further increasing efficiency.
Third, SANA-Sprint integrates ControlNet for interactive image generation in real-time. This allows for immediate visual feedback for user interaction.
Performance and Speed
SANA-Sprint sets new standards in the relationship between speed and quality. With just one step, it achieves an FID score of 7.59 and a GenEval score of 0.74. This surpasses FLUX-fast (7.94 FID / 0.71 GenEval) while being ten times faster (0.1 seconds vs. 1.1 seconds on an H100 GPU). For images with a resolution of 1024 x 1024, SANA-Sprint achieves a latency of 0.1 seconds (T2I) and 0.25 seconds (ControlNet) on an H100 GPU. On an RTX 4090 GPU, the latency is 0.31 seconds (T2I). These results underscore the exceptional efficiency and potential of SANA-Sprint for AI-powered consumer applications (AIPC).
Outlook
The developers of SANA-Sprint plan to release the code and pre-trained models. This will allow the research community to build on and further develop this promising technology. The high speed and quality of the generated images open up new possibilities for applications in various fields, from the creation of marketing materials to the development of video games.
Especially for companies like Mindverse, which specialize in AI-powered content creation, SANA-Sprint offers the potential to revolutionize image generation. Integrating SANA-Sprint into platforms like Mindverse could significantly accelerate and simplify the creation of high-quality imagery.
Bibliographie: Chen, J., Xue, S., Zhao, Y., Yu, J., Paul, S., Chen, J., Cai, H., Xie, E., & Han, S. (2025). SANA-Sprint: One-Step Diffusion with Continuous-Time Consistency Distillation. *arXiv preprint arXiv:2503.09641*. Unknown. (2023). *arxiv:2311.18828*. Unknown. (2025). *arxiv:2502.07579*. Unknown. (2024). *neurips.cc/virtual/2024/poster/93608*. Unknown. (2024). *arxiv.org/html/2410.11081v1*. Unknown. (2021). *ddd.uab.cat/pub/tesis/2021/hdl_10803_668629/cbm1de1.pdf*. Unknown. (2012). *registrar.vanderbilt.edu/documents/2012_2013_Graduate_Catalog.pdf*. Unknown. (n.d.). *openreview.net/forum?id=ogk236hsJM*. Unknown. (2024). *ersnet.org/wp-content/uploads/2024/07/Congress-Programme-2024-2507-02.pdf*.