Multimodal Language Models Enhance Single Cell Analysis
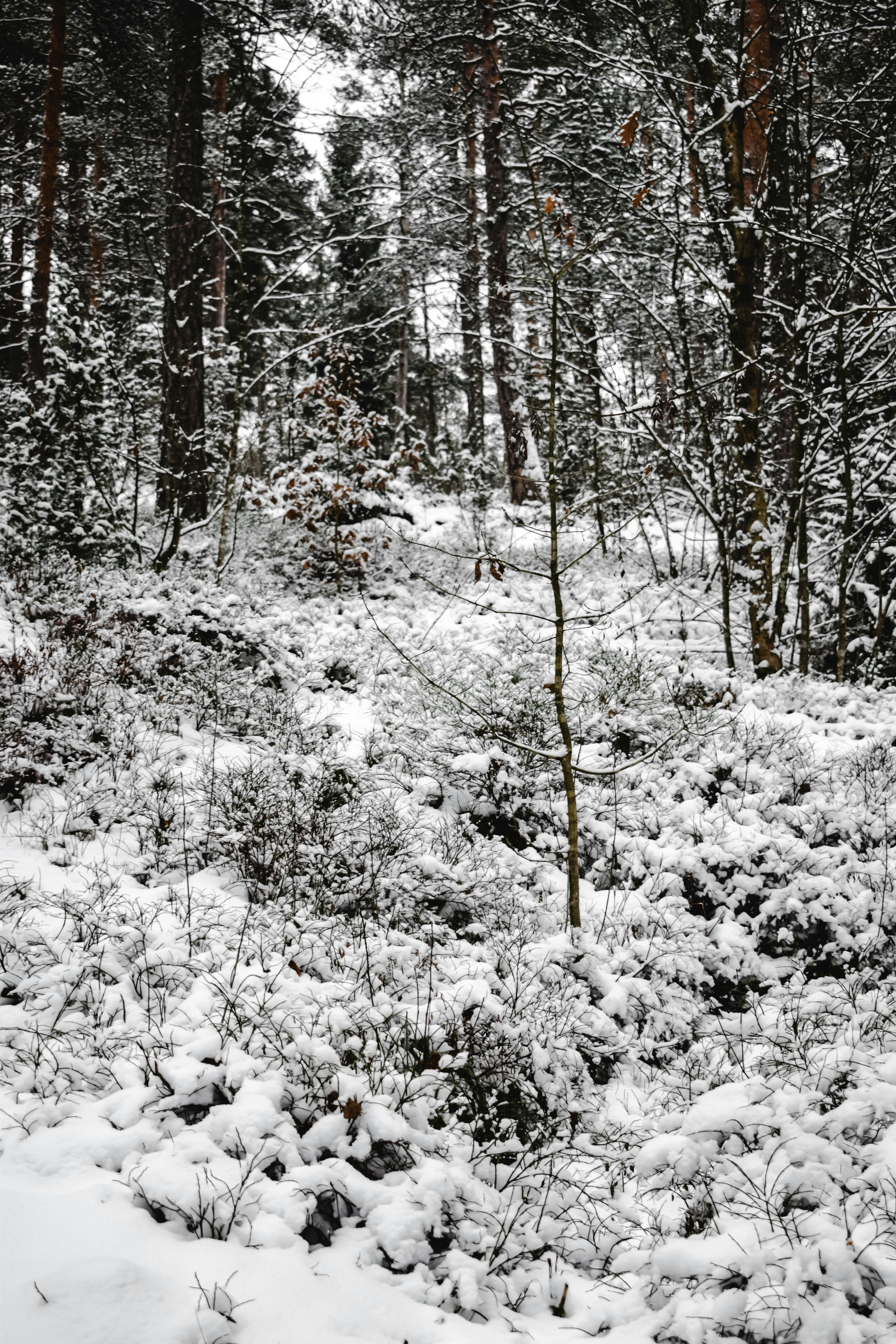
Top post
Revolution in Single-Cell Analysis: Multimodal Language Models Open New Possibilities
Single-cell analysis has made enormous progress in recent years and revolutionized our understanding of biological processes at the cellular level. A promising approach to further improve these analysis methods lies in the application of artificial intelligence, particularly multimodal language models. These models are capable of processing and integrating both text data and cell information, leading to new insights and improved predictions.
Challenges and Opportunities of Multimodal Language Models
Previous approaches to analyzing single-cell data using AI encountered various challenges. Traditional language models, trained on text data, could not process the complex information from RNA sequencing data. Conversely, models specifically developed for cell data lacked the ability to interpret free text, which limited their use in multimodal tasks. The integration of both modalities often led to information loss or insufficiently pre-trained models, resulting in suboptimal results.
Multimodal language models offer the opportunity to overcome these hurdles. They enable the joint modeling of cell and text data and promote knowledge exchange between the modalities. This opens up new possibilities for the analysis and interpretation of single-cell data, such as automated cell type annotation, the generation of cell descriptions, and the prediction of cell behavior.
scMMGPT: A Promising Approach
One example of such a multimodal language model is scMMGPT (Single-Cell MultiModal Generative Pre-trained Transformer). This model integrates state-of-the-art cell and text language models and utilizes dedicated cross-modal projectors to bridge the gap between the text and cell modalities. scMMGPT was pre-trained on a comprehensive dataset of 27 million cells – the largest dataset to date for multimodal cell-text language models.
This extensive training allows scMMGPT to achieve outstanding results in joint cell-text tasks. For example, a relative improvement of 84% in textual discrepancy for the generation of cell descriptions was achieved. scMMGPT also achieved convincing results in cell type annotation with a 20.5% higher accuracy. Furthermore, the k-NN accuracy for text-conditioned pseudo-cell generation improved by 4% compared to previous approaches.
Outlook: Potential for Research and Development
The development of multimodal language models like scMMGPT represents a significant advance in single-cell analysis. By integrating cell and text data, new possibilities are opened up for biomedical research and the development of personalized therapies. The ability to better understand complex biological processes at the cellular level contributes to earlier diagnosis of diseases, the development of more individualized treatment strategies, and the improvement of drug efficacy.
The continued development and application of multimodal language models thus promises a deeper understanding of life processes and opens new avenues for medical research and development.
Bibliography: Shi, Y., Yang, J., Li, S., Fang, J., Wang, X., Liu, Z., & Zhang, Y. (2025). Multimodal Language Modeling for High-Accuracy Single Cell Transcriptomics Analysis and Generation. *arXiv preprint arXiv:2503.09427*. Hugging Face Papers. https://huggingface.co/papers Ma, S., et al. (2025). Deep parametric inference for single-cell multimodal omics data analysis. *Nature Communications, 16*(1), 4854. Lopez, R., Regier, J., Cole, M. B., Jordan, M. I., & Yosef, N. (2024). Deep generative modeling for single-cell transcriptomics. *Nature Biotechnology, 42*(2), 206-216. ChatPaper. https://chatpaper.com/chatpaper/fr?id=5&date=1741795200&page=1 Awesome Deep Learning Single Cell Papers. https://github.com/OmicsML/awesome-deep-learning-single-cell-papers Xu, C., et al. (2024). scGPT: Towards Building a Foundation Model for Single-Cell Multi-omics using Generative AI. *arXiv preprint arXiv:2412.03614*. Li, Y., et al. (2024). scBERT as a Large Language Model for Single-Cell RNA Sequencing Data. *bioRxiv*. Nurk, S., et al. (2025). The European Nucleotide Archive in 2025. *Nucleic Acids Research, 53*(D1), D886–D897. Wang, X., et al. (2024). scFormer: Pre-trained Language Model for Single-cell Transcriptomics using Transformer. *arXiv preprint arXiv:2407.09811*.