Early Development of Reflection in Language Models
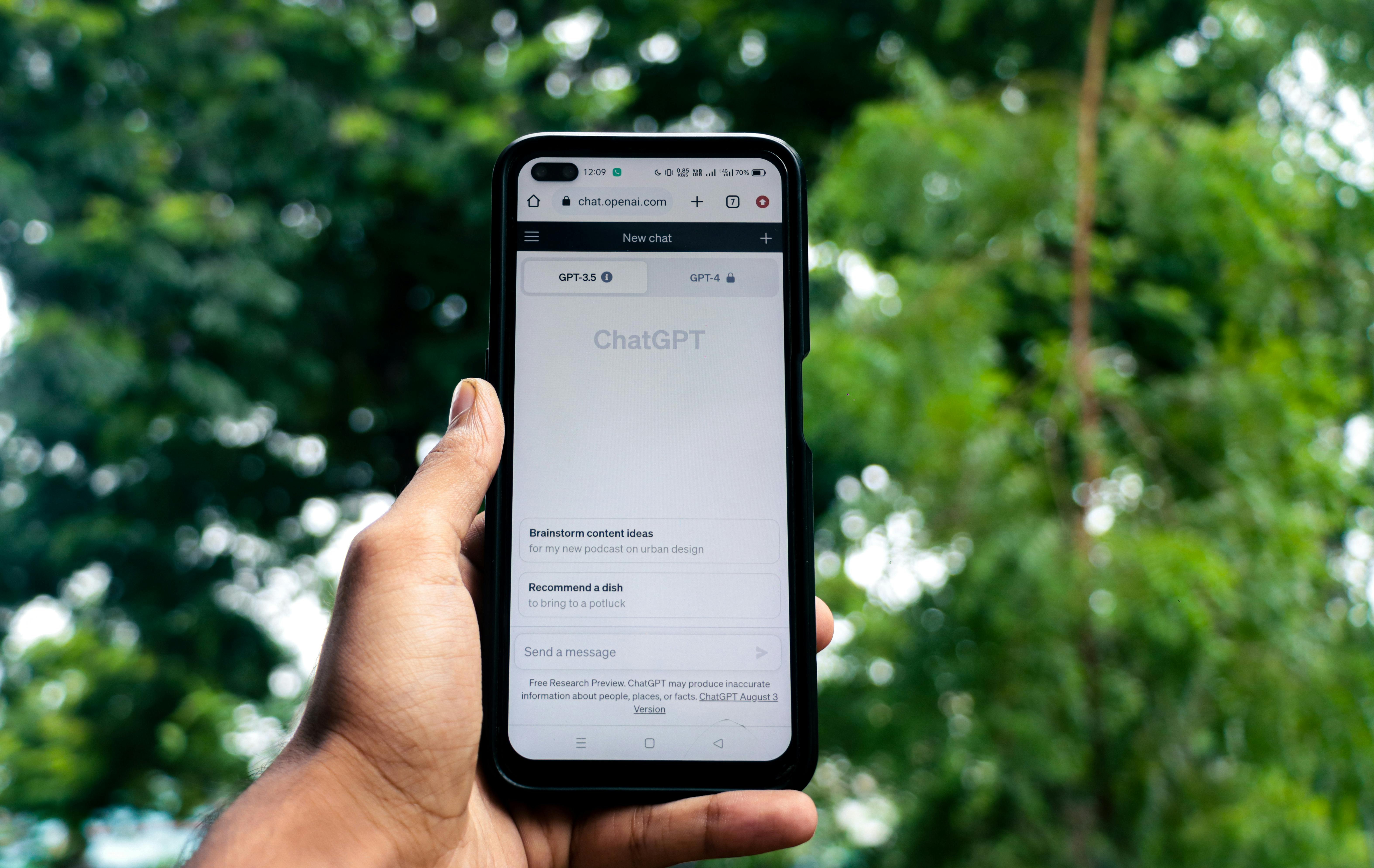
Top post
Reflection in Focus: New Insights into the Early Development of Language Models
The ability of language models to reflect on and correct their own thought processes is considered key to solving complex problems. Previously, research mainly focused on developing this ability within the framework of reinforcement learning. However, new findings suggest that this ability emerges and develops much earlier, namely during pre-training.
A recent study examines this aspect in more detail. By inserting deliberate errors into so-called "chains-of-thought," the study analyzes whether the model still arrives at the correct solution by recognizing and correcting the errors. The results show that this ability for self-correction appears early in the training process and continuously improves.
An example: The OLMo2-7B model, trained with 4 trillion tokens, already demonstrated the ability for self-correction in six self-reflection tasks. This observation suggests that the capacity for reflection is not exclusively a product of reinforcement learning, but is already established during pre-training.
The Importance of Pre-Training
The pre-training of language models forms the foundation for their later abilities. In this phase, the models are fed with massive amounts of data and learn to recognize statistical relationships between words and sentences. This fundamental language competence forms the basis for more complex abilities such as reflection.
The new findings underscore the importance of pre-training for the development of powerful AI systems. A deeper understanding of the processes that occur during pre-training could contribute to more targeted control of the development of language models and further improve their capabilities.
Outlook and Implications for AI Research
The discovery that the capacity for reflection already emerges during pre-training opens new perspectives for AI research. Future studies could focus on identifying the factors that influence the development of this ability in pre-training. This could lead to new training methods that more specifically promote the reflective capacity of language models.
For companies like Mindverse, which specialize in the development of AI solutions, these findings are of particular interest. A deeper understanding of the learning processes of language models enables the development of even more powerful and robust AI systems, be it in the form of chatbots, voicebots, AI search engines, or knowledge databases.
The research results highlight the enormous potential of language models and open up exciting possibilities for the future of artificial intelligence. The continuous further development and optimization of pre-training could be the key to even more powerful and versatile AI systems.
Bibliographie: https://arxiv.org/abs/2504.04022 https://x.com/iScienceLuvr/status/1909537059834151383 https://www.researchgate.net/publication/363322299_Rethinking_reflective_practice_John_Boyd's_OODA_loop_as_an_alternative_to_Kolb http://paperreading.club/page?id=297637 https://journals.sagepub.com/doi/full/10.1177/00224871231188702 https://openreview.net/forum?id=a06UO11IrQ https://arxiv.org/abs/2006.06882 https://www.researchgate.net/publication/357863985_Rethinking_reflection https://www.tandfonline.com/doi/full/10.1080/00131881.2024.2346088 https://www.sciencedirect.com/science/article/pii/S1472811722001057