DreamRenderer Improves Control Over Multi-Instance Image Synthesis
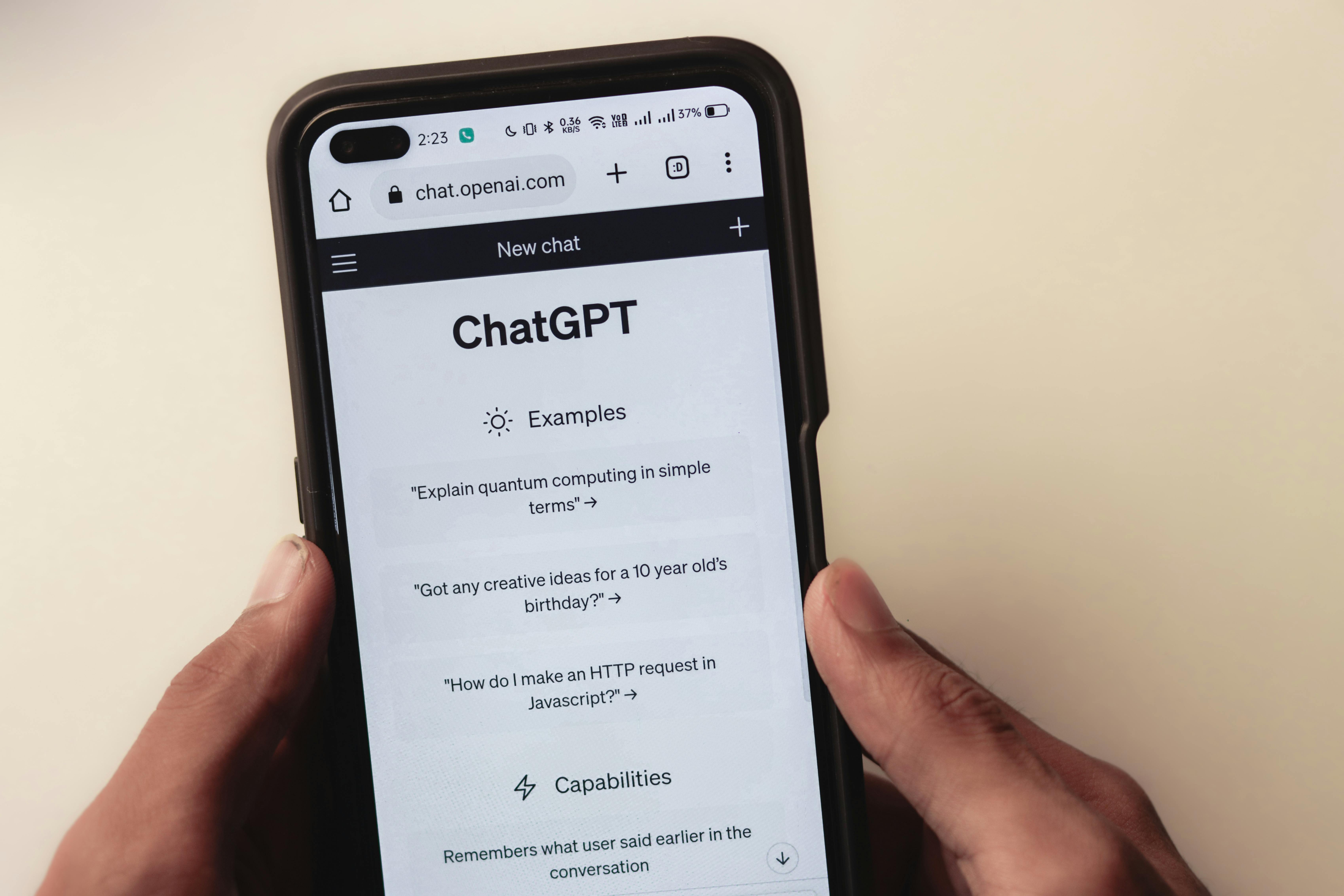
Top post
Precise Image Synthesis with DreamRenderer: More Control over Multiple Instances
Precisely controlling content in image synthesis, particularly with multiple instances or regions within an image, presents a challenge for existing AI models. Even state-of-the-art methods like FLUX and 3DIS struggle with issues such as the unwanted transfer of attributes between different instances, limiting the user's creative control.
DreamRenderer, a new, training-free approach building upon the FLUX model, promises a remedy. This innovative approach allows users to control the content of each individual instance via bounding boxes or masks while maintaining the visual harmony of the overall image. DreamRenderer requires no additional training and can thus be easily integrated into existing workflows.
The Innovations of DreamRenderer
Two core innovations distinguish DreamRenderer. First, the "Bridge Image Tokens for Hard Text Attribute Binding". This method uses replicated image tokens as bridge tokens to ensure that the T5 text embeddings, which are pre-trained exclusively on text data, bind the correct visual attributes for each instance during joint attention. This prevents the unwanted "leakage" of attributes between individual instances.
Second, the "Hard Image Attribute Binding", which is selectively applied only to crucial layers. Through an analysis of the FLUX model, the layers responsible for rendering instance attributes were identified. Hard Image Attribute Binding is applied only in these layers, while a "Soft Binding" is used in the remaining layers. This approach ensures precise control over attributes while preserving image quality.
Evaluation and Results
The evaluation of DreamRenderer on the COCO-POS and COCO-MIG benchmarks shows a significant improvement in the "Image Success Ratio" of 17.7% compared to FLUX. The performance of layout-to-image models like GLIGEN and 3DIS could also be increased by up to 26.8% through the integration of DreamRenderer. These results underscore the potential of DreamRenderer for precise and controlled image synthesis.
The ability to precisely control multiple instances within an image opens up new possibilities for creative applications and design processes. From the creation of complex compositions to the targeted manipulation of individual image elements, DreamRenderer offers a promising tool for the future of image generation.
Applications and Future Perspectives
The precise control of image elements using AI opens up diverse application possibilities in various fields. In design, complex compositions and product visualizations could be created more efficiently. In art, artists could explore new forms of expression and implement their creative visions with a higher degree of control. DreamRenderer also offers a valuable tool for research and development in the investigation and further development of AI-based image synthesis methods.
The further development of DreamRenderer and similar approaches promises even more precise and intuitive control of AI-generated images. Future research could focus on improving user-friendliness, expanding the supported image types, and integrating further control mechanisms. The combination of powerful AI models with intuitive user interfaces will make image synthesis accessible to a wider audience and open up new creative possibilities.
Bibliographie: Zhou, D., Li, M., Yang, Z., & Yang, Y. (2024). DreamRenderer: Taming Multi-Instance Attribute Control in Large-Scale Text-to-Image Models. *arXiv preprint arXiv:2503.12885*. Zhou, K., Zhang, W., Wang, H., Wei, F., Loy, C. C., & Lin, D. (2024). MIGC: Multi-Instance Generation Controller for Text-to-Image Synthesis. *Proceedings of the IEEE/CVF Conference on Computer Vision and Pattern Recognition*, 16742–16752. AlonzoLeeeooo. (n.d.). *Awesome-text-to-image-studies*. GitHub. Retrieved from https://github.com/AlonzoLeeeooo/awesome-text-to-image-studies Su, Y., He, Y., Song, Y., & Guibas, L. J. (2024). Text-to-Image Editing by Image Information Removal. *arXiv preprint arXiv:2402.05408*. Shakespeare and Comedy (Bloom’s Modern Critical Interpretations). (2010). Infobase Publishing. Chris2D. (n.d.). *High-Frequency Word List*. Retrieved from https://www.cs.unm.edu/~chris2d/papers/freq2.txt Chris2D. (n.d.). *Words of Very High Frequency*. Retrieved from https://www.cs.unm.edu/~chris2d/papers/freq.txt Harrell, A. M. (2014). *The Louisiana Purchase Exposition and its Impact on the Dissemination of Modern Architecture in the United States* (Doctoral dissertation, Louisiana State University and Agricultural and Mechanical College).