R1-Onevision: A New Multimodal Reasoning Model and Benchmark
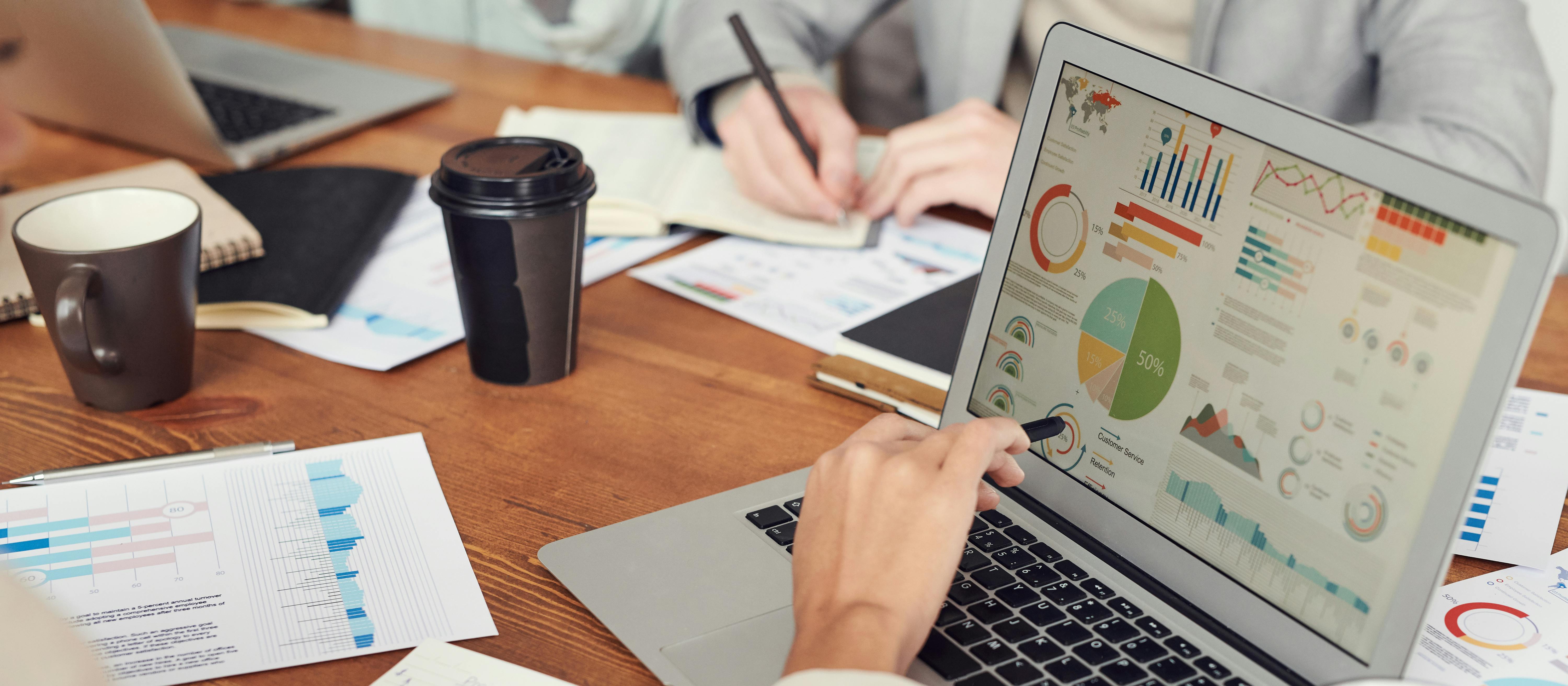
Top post
Multimodal Reasoning: R1-Onevision – A New Approach for Enhanced Visual-Language Reasoning
The rapid development in the field of Artificial Intelligence has led to impressive advances in machine reasoning in recent years. Large Language Models (LLMs) demonstrate remarkable abilities to solve complex text-based tasks and draw conclusions. However, the integration of visual information and its connection with text – so-called multimodal reasoning – continues to pose significant challenges for AI research.
Existing visual-language models often struggle to effectively analyze visual content and incorporate it into logical reasoning. This leads to suboptimal results in complex tasks that require a deeper understanding of both image and text. Furthermore, the lack of comprehensive benchmarks makes it difficult to precisely evaluate multimodal reasoning capabilities.
R1-Onevision: A Promising Approach
Against this backdrop, R1-Onevision presents itself as a promising approach to bridge the gap between visual perception and deep reasoning. The model is based on an innovative cross-modal reasoning pipeline that transforms images into formal text representations. This transformation enables precise language-based reasoning by converting visual information into a format understandable for LLMs.
To enable the training and evaluation of R1-Onevision, a dedicated dataset was developed. This dataset, also called R1-Onevision, offers detailed, step-by-step annotations for multimodal reasoning in various domains. By providing detailed explanations of the reasoning steps, the model is trained to grasp complex relationships between image and text.
Training and Evaluation with R1-Onevision-Bench
The training of the R1-Onevision model is carried out through supervised learning and reinforcement learning. This combination allows the model to both learn from existing data and improve its abilities through interaction and feedback. This promotes advanced reasoning skills and robust generalization to new, unseen data.
For a comprehensive evaluation of multimodal reasoning capabilities, R1-Onevision-Bench was developed, a benchmark oriented towards human educational stages. This benchmark includes exams from secondary school to university level and beyond, to test the model's performance at different levels of difficulty.
Results and Outlook
Initial results show that R1-Onevision achieves compelling performance compared to other models like GPT-4o and Qwen2.5-VL, surpassing the current state-of-the-art in several challenging multimodal reasoning benchmarks. The ability to effectively integrate visual information into the reasoning process opens up new possibilities for the application of AI in areas such as image captioning, medical diagnostics, and robotics.
Research in the field of multimodal reasoning is far from complete. However, R1-Onevision represents an important step towards an AI capable of more comprehensively understanding the world around us and solving more complex problems. Future research will focus on further improving the robustness and generalization ability of such models and exploring new application areas.
Bibliography: - https://huggingface.co/papers/2503.10615 - https://huggingface.co/papers - https://arxiv.org/abs/2503.06749 - https://chatpaper.com/chatpaper/fr?id=4&date=1741881600&page=1 - https://chatpaper.com/chatpaper/zh-CN?id=4&date=1741881600&page=1 - https://github.com/gabrielchua/daily-ai-papers - https://paperswithcode.com/paper/r1-onevision-an-open-source-multimodal-large - https://arxiv.org/html/2503.09567v1 - https://github.com/joheras/Lecturas - https://mediatum.ub.tum.de/doc/1616775/ln730px9m4l1lwepugycjagj5.Florian_Walter_Advanced_Embodied_Learning.pdf