Multimodal Chain-of-Thought Reasoning: Advancing AI with Combined Modalities
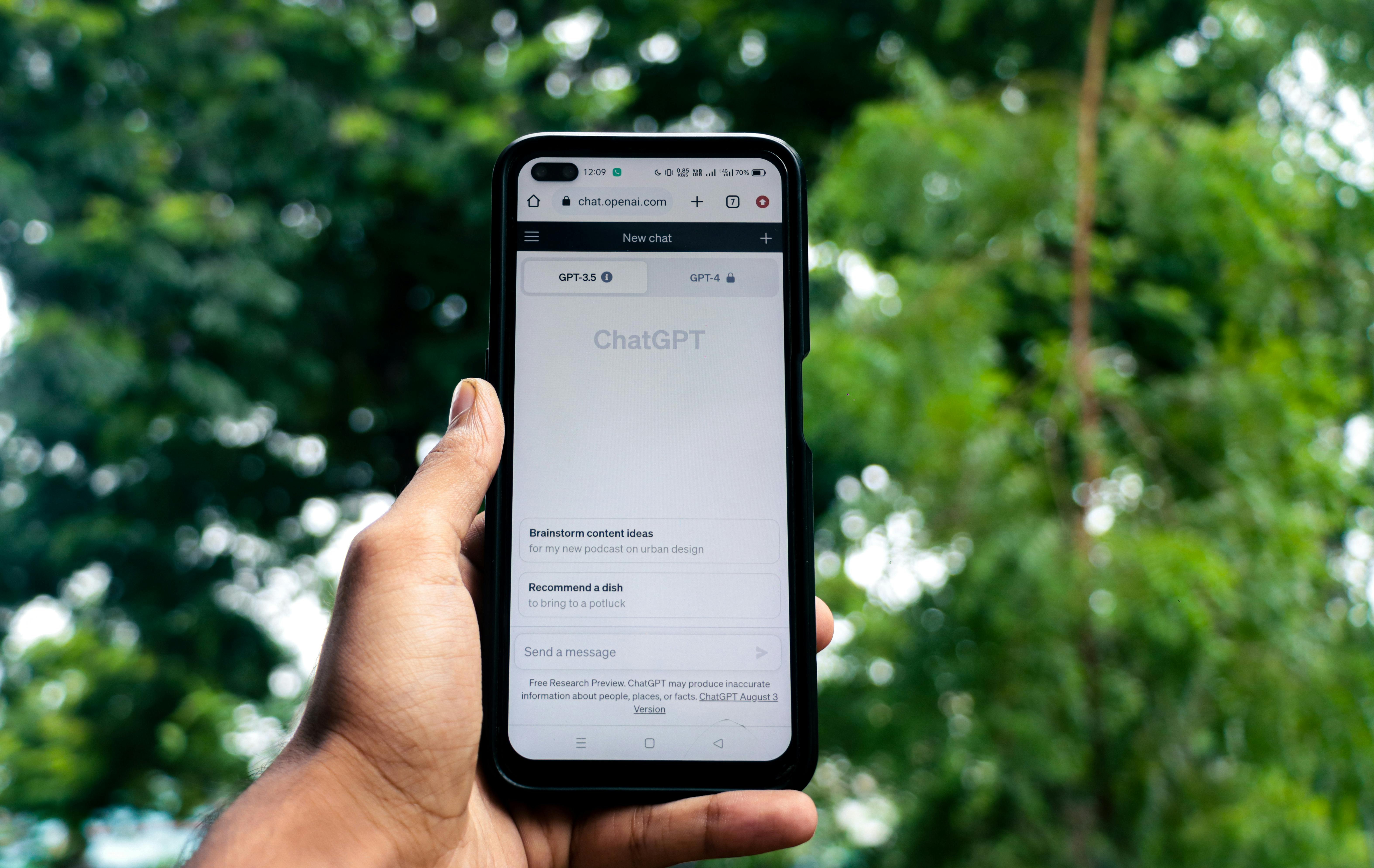
Top post
Multimodal Thinking: Chains of Reasoning in a Multimodal World
Artificial intelligence (AI) is rapidly evolving, and a particularly exciting area is multimodal Chain-of-Thought Reasoning (MCoT). MCoT allows AI systems to combine information from various sources, such as images, texts, and audio, and to draw step-by-step, human-like conclusions. This capability opens up new possibilities for AI applications in a wide variety of fields.
What is MCoT?
MCoT builds on the concept of Chain-of-Thought Reasoning (CoT), where AI models reveal their thought processes in a series of intermediate steps. In contrast to traditional black-box models, which only provide a final answer, CoT models offer insights into their reasoning. MCoT extends this principle to multimodal data by combining information from different modalities to draw more complex conclusions. An example of this would be an AI system that analyzes an image, reads accompanying text, and then answers questions about the image content, transparently presenting its thought steps.
Methods and Approaches
Research in the field of MCoT has produced various methods to address the challenges of multimodal reasoning. These include:
Rationale Construction: Here, the model constructs a justification for its conclusion by linking the different modalities. Multimodal Thought: This method integrates information from different modalities into each individual thought step. Test-Time Scaling: This uses additional information from other modalities during testing to improve the accuracy of the conclusions. Further approaches deal with the optimization of thought processes, the integration of knowledge graphs, and the development of new architectures for multimodal models.
Areas of Application
The potential of MCoT is enormous and extends across various application areas:
Robotics: MCoT enables robots to better understand their environment and perform more complex tasks. Healthcare: AI systems can analyze medical images and combine them with patient data to support diagnoses. Autonomous Driving: MCoT helps autonomous vehicles interpret complex traffic situations and make safe decisions. Multimodal Generation: AI models can generate texts, images, and other media content that are coherent and context-related.
Challenges and Future Perspectives
Despite the promising advances in the field of MCoT, there are still some challenges to overcome:
Generalization: MCoT models must be able to transfer their knowledge to new, unknown situations. Dynamic Chain Optimization: The length and complexity of the thought chains must be adapted to the respective task. Hallucinations: AI models can sometimes generate false or misleading information. Safety: It is important to ensure that MCoT models are robust and reliable, especially in safety-critical applications.
Future research in the field of MCoT will focus on these challenges and develop new methods to further improve the performance and reliability of multimodal AI systems. The development of robust benchmarks and datasets will also play an important role in measuring and promoting progress in this area.
Bibliography: Wu, Shengqiong, et al. "Multimodal Chain-of-Thought Reasoning: A Comprehensive Survey." arXiv preprint arXiv:2309.15402 (2023). Zhang, Yuecheng, et al. "Multimodal chain-of-thought reasoning in language models." arXiv preprint arXiv:2302.00923 (2023). Yao, Shunyu, et al. "REACT: Synergizing reasoning and acting in language models." arXiv preprint arXiv:2210.03629 (2022). Driess, Danny, et al. "Palm-e: An embodied multimodal language model." arXiv preprint arXiv:2303.03378 (2023).