Multimodal Language Models for Image Quality and Aesthetics Assessment
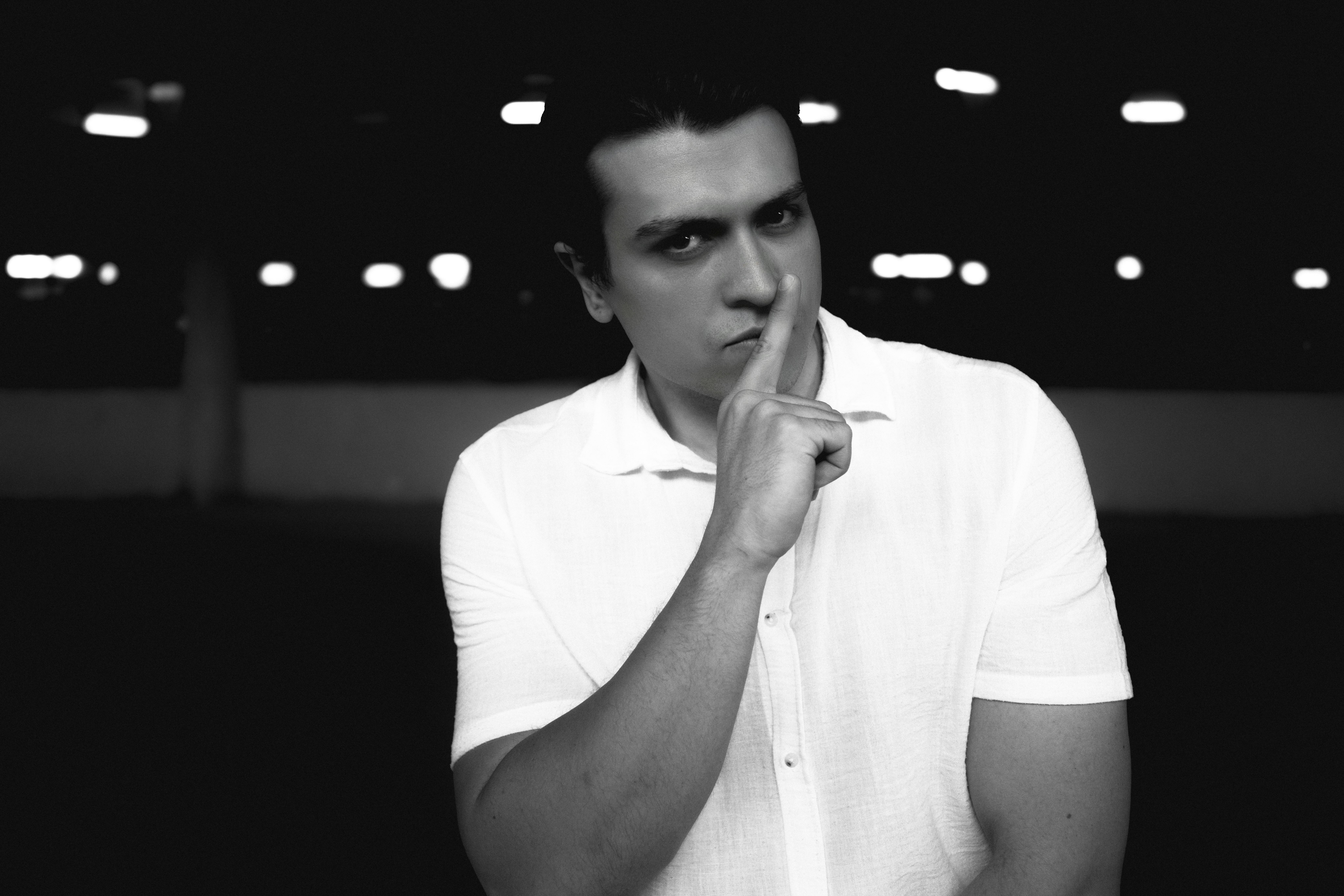
Top post
Multimodal Language Models for Evaluating Image Quality and Aesthetics
The explosive growth of User-Generated Content (UGC) on the internet, especially images, presents new challenges for evaluating this content. Assessing image quality and aesthetics is of crucial importance. Multimodal Large Language Models (MLLMs) show promising results in the fields of Image Quality Assessment (IQA) and Image Aesthetic Assessment (IAA). Despite this progress, difficulties remain in effectively evaluating UGC images. Two central challenges are the inadequate capture of multifaceted human perception by a single score and the question of how MLLMs can be used to output numerical ratings, such as Mean Opinion Scores (MOS).
The RealQA Dataset and the Prediction of Numerical Ratings
To address these challenges, the RealQA (Realistic image Quality and Aesthetic) dataset was developed. It comprises 14,715 UGC images, each annotated with 10 fine-grained attributes. These attributes cover three levels: Low-Level (e.g., image sharpness), Mid-Level (e.g., subject integrity), and High-Level (e.g., composition). Through detailed annotation, RealQA enables a more nuanced evaluation of image quality and aesthetics.
Another focus of research is the effective prediction of numerical ratings by MLLMs. Surprisingly, the next-token paradigm can achieve state-of-the-art results by predicting only two additional significant digits. In combination with Chain-of-Thought (CoT) and the learned fine-grained attributes, the proposed method outperforms existing methods on five public datasets for IQA and IAA. Moreover, it shows improved interpretability and strong zero-shot generalization for Video Quality Assessment (VQA).
The Importance of Fine-Grained Attributes
The use of fine-grained attributes allows for a more differentiated evaluation of images and contributes to the interpretability of the results. Instead of providing just a single score, MLLMs can offer detailed insights into the strengths and weaknesses of an image by considering various attributes. This is particularly important for analyzing UGC images, as they can vary greatly in quality and aesthetics.
Future Perspectives and Applications
Research in the field of automated image evaluation with MLLMs is dynamic and promising. The development of new datasets and methods contributes to the continuous improvement of the accuracy and interpretability of results. The application possibilities are diverse and range from quality control in photography and video production to automated content moderation in social media. The combination of MLLMs with fine-grained attributes and advanced techniques like CoT opens new avenues for a comprehensive and differentiated evaluation of images and videos.
The publication of the code and the RealQA dataset will advance further research in this area and enable the development of new applications. Especially for companies like Mindverse, which specialize in AI-powered content creation and analysis, these developments offer new opportunities to optimize their products and services.
Bibliography: https://arxiv.org/abs/2503.06141 https://arxiv.org/html/2503.06141v1 http://paperreading.club/page?id=290498 https://2024.emnlp.org/program/accepted_findings/ https://github.com/gabrielchua/daily-ai-papers https://openreview.net/forum?id=IRXyPm9IPW&referrer=%5Bthe%20profile%20of%20Jing%20Xiong%5D(%2Fprofile%3Fid%3D~Jing_Xiong4) https://nips.cc/virtual/2024/papers.html https://paperswithcode.com/author/rita-cucchiara https://www.researchgate.net/publication/381668798_EvalAlign_Evaluating_Text-to-Image_Models_through_Precision_Alignment_of_Multimodal_Large_Models_with_Supervised_Fine-Tuning_to_Human_Annotations