VisualWebInstruct Leverages Web Search to Generate Multimodal Instruction Data
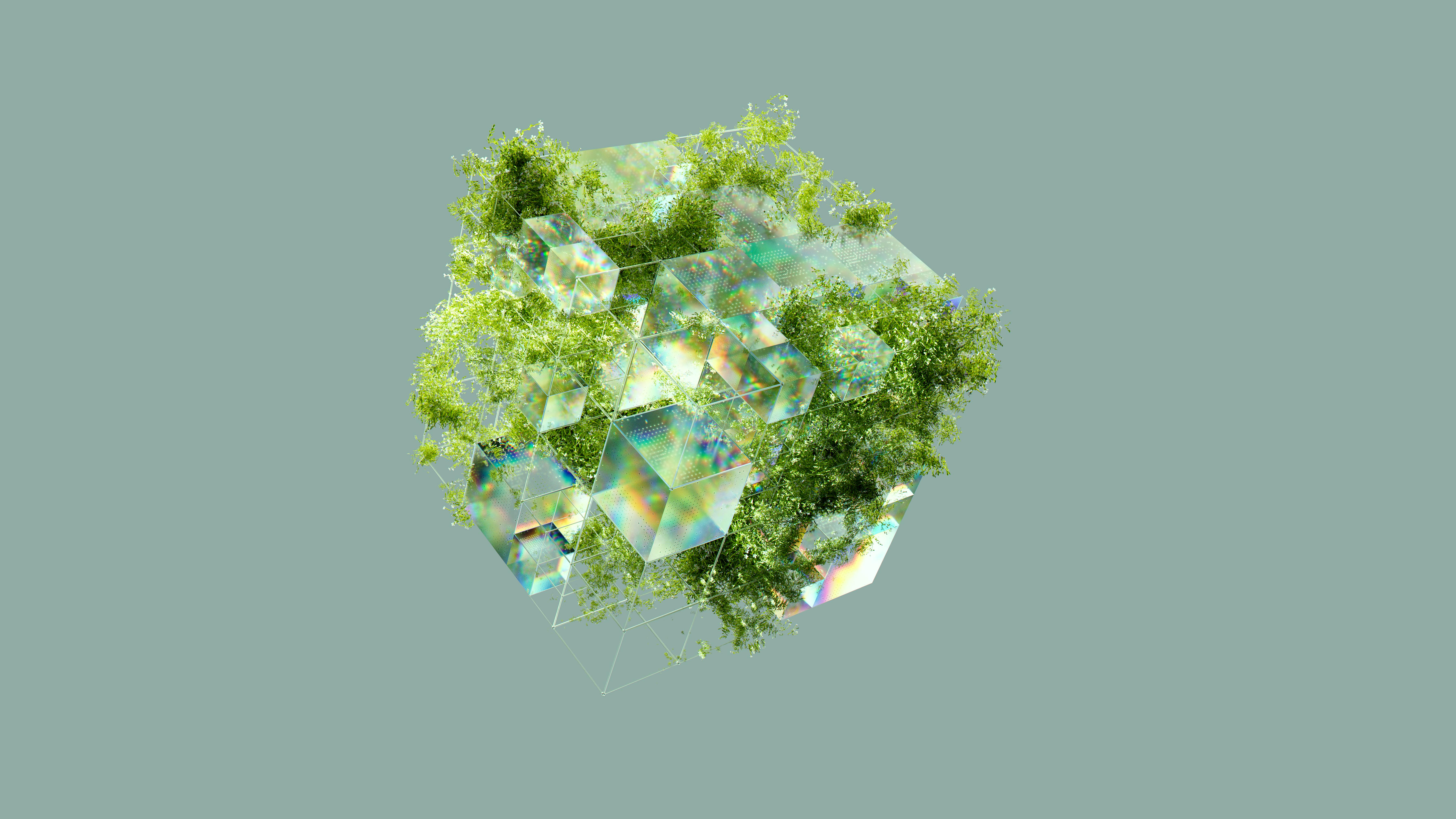
Top post
Large-Scale Multimodal Instruction Data: VisualWebInstruct Leverages Web Search
Vision-Language Models (VLMs) have made remarkable progress in perception-oriented tasks in recent years. However, their abilities in complex reasoning, such as mathematical deduction or understanding physical relationships, often fall short of expectations. A primary reason for this is the lack of high-quality and diverse training data specifically tailored to such tasks. A new approach called VisualWebInstruct promises a remedy by leveraging the power of web search to generate large amounts of multimodal instruction data.
The team behind VisualWebInstruct pursues an innovative approach to data collection. Starting with a carefully selected set of 30,000 images, they use Google Image Search to identify web pages containing similar images. The HTML content of over 700,000 unique URLs is collected and subsequently subjected to multi-stage processing. This includes extracting relevant information, filtering unsuitable content, and finally synthesizing question-answer pairs. The result is a comprehensive dataset with approximately 900,000 entries, 40% of which are visual question-answer pairs and the remaining 60% are text-based question-answer pairs.
The effectiveness of VisualWebInstruct has been demonstrated by fine-tuning various VLMs. Models trained with this dataset show significant improvements in various benchmarks. For example, models trained with Llava-OV-mid achieved increases of 10-20%, while MAmmoTH-VL models gained 5%. Particularly impressive is the performance of the optimized model MAmmoTH-VL2, which achieves top performance in the 10-billion parameter class on MMMU-Pro-std (40.7%), MathVerse (42.6%), and DynaMath (55.7%). These results underscore the potential of VisualWebInstruct to significantly improve the reasoning capabilities of VLMs for complex multimodal tasks.
The Significance of VisualWebInstruct for the Future of AI
The development of VisualWebInstruct represents an important step towards more powerful and versatile VLMs. By leveraging the vast amounts of data available on the internet, this approach opens up new possibilities for training AI models. The ability to extract contextual information from images and texts and draw complex conclusions from it is crucial for the development of AI systems with human-like cognitive abilities. VisualWebInstruct helps to close this gap and paves the way for future applications in areas such as education, research, and industry.
For Mindverse, a German company specializing in customized AI solutions, such advancements in AI research are of great importance. The development of chatbots, voicebots, AI search engines, and knowledge systems benefits directly from more powerful VLMs. VisualWebInstruct and similar approaches make it possible to further increase the quality and efficiency of these systems and open up new possibilities for innovative applications.
VisualWebInstruct and the Challenges of the Future
Despite the promising results, using web data for AI training also presents challenges. The quality of information available on the internet is not always guaranteed, and there is a risk of biases and misinformation entering the training data. The developers of VisualWebInstruct are aware of this issue and have taken steps to ensure data quality through filtering and validation. However, further research in this area is essential to develop robust and reliable AI systems that can also handle the uncertainties of the internet.
Bibliographie: Jia, Yiming, et al. "VisualWebInstruct: Scaling up Multimodal Instruction Data through Web Search." *arXiv preprint arXiv:2503.10582* (2025). https://huggingface.co/papers/2503.10582 https://huggingface.co/papers https://github.com/TIGER-AI-Lab https://arxiv.org/html/2404.05955v1 https://arxiv.org/html/2305.11854v4 https://dl.acm.org/doi/10.1145/3616855.3635753 https://www.researchgate.net/publication/370938268_Multimodal_Web_Navigation_with_Instruction-Finetuned_Foundation_Models/download https://www.sciencedirect.com/science/article/abs/pii/S092523122401909X https://openreview.net/forum?id=IIsTO4P3Ag https://www.researchgate.net/publication/388421847_Eagle_2_Building_Post-Training_Data_Strategies_from_Scratch_for_Frontier_Vision-Language_Models