Large Reasoning Models Transforming Machine Translation
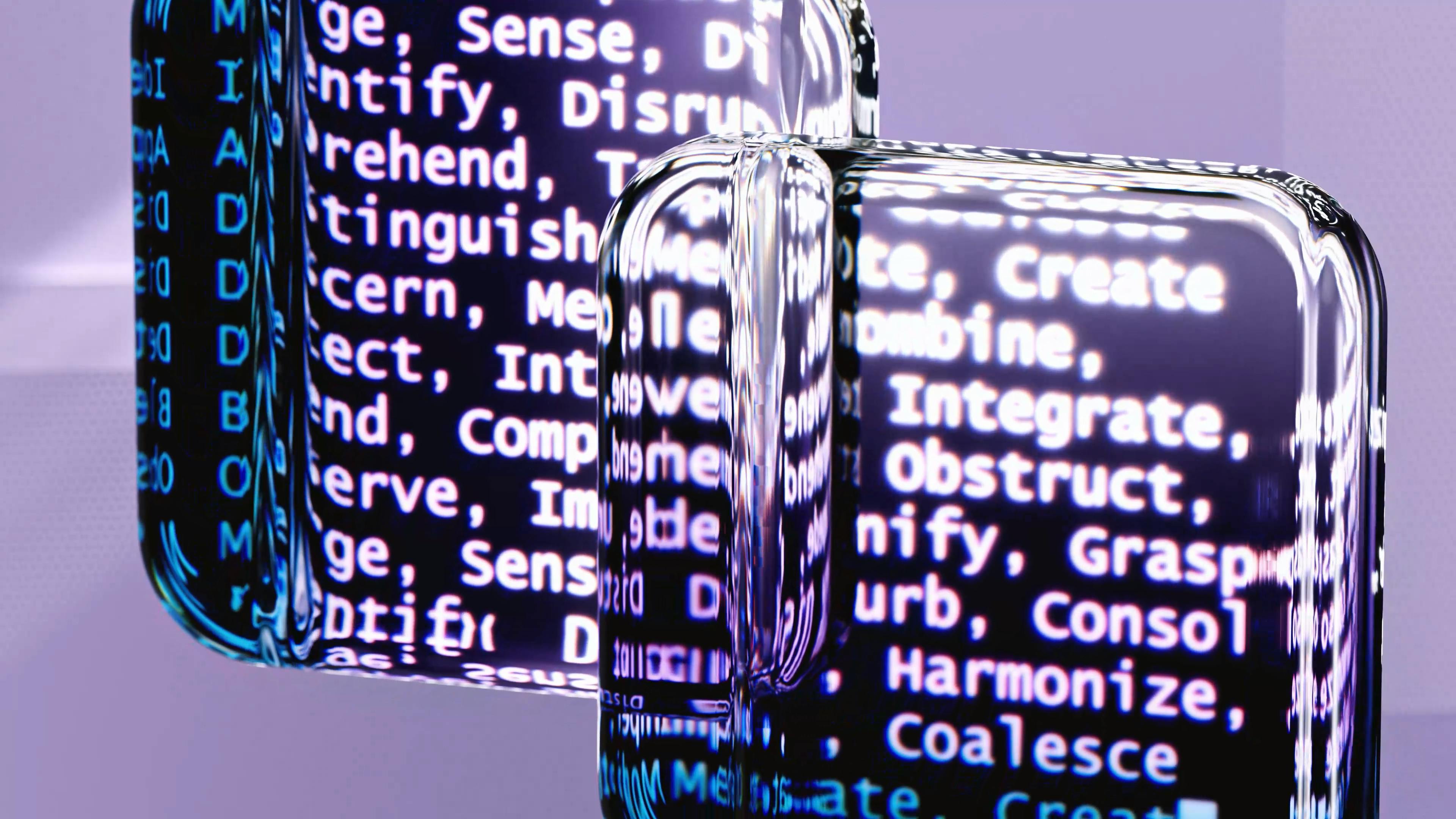
Top post
Machine Translation in Transition: Large Reasoning Models Open Up New Possibilities
Machine translation (MT) is currently undergoing a fundamental shift, driven by advances in large language models (LLMs). In particular, so-called Large Reasoning Models (LRMs), which simulate thinking processes modeled on human thought (Chain-of-Thought, CoT), promise a new era of MT. These models no longer view translation as mere text conversion, but as a dynamic reasoning task that requires contextual, cultural, and linguistic understanding.
Three Fundamental Changes through LRMs
LRMs lead to three central changes in the field of MT:
First, they enable contextual coherence. By explicitly reasoning about complex, cross-sentence relationships – or even in the absence of context – LRMs can resolve ambiguities and maintain discourse structure. This leads to more fluent and accurate translations that consider the overall context.
Second, LRMs promote cultural intentionality. The models can infer the speaker's intention, the audience's expectations, and sociolinguistic norms, and adapt their output accordingly. This enables translations that are culturally appropriate and target-group oriented.
Third, LRMs are characterized by self-reflection. During the translation process, they can detect and correct potential errors, especially with noisy input data. This ability for self-correction increases the robustness of the translation systems.
Application Examples and New Phenomena
The application of LRMs opens up new possibilities in various translation scenarios, including stylized translations, document-based translations, and multimodal translations. Empirical examples demonstrate the superiority of LRMs in these areas. Furthermore, LRMs enable new phenomena such as auto-pivot translation, where an intermediate language is used for translation.
Challenges and Outlook
Despite the promising advances, challenges remain. These include the risk of over-localization, where cultural adaptations go too far, as well as the computational intensity and associated inefficiency of the inference processes. Optimizing inference speed is an important research focus.
Overall, LRMs are fundamentally changing the role of translation systems. They are evolving from pure text converters to multilingual cognitive agents that can think beyond the mere text. This paradigm shift opens new perspectives for MT and encourages thinking beyond traditional translation scenarios. Future research will focus on exploiting the full potential of LRMs and addressing the existing challenges.
Bibliography: Liu, S., Lyu, C., Wu, M., Wang, L., Luo, W., & Zhang, K. (2025). New Trends for Modern Machine Translation with Large Reasoning Models. *arXiv preprint arXiv:2503.10351*. Hugging Face Papers. https://huggingface.co/papers Lyu, C., Liu, S., Wu, M., Wang, L., Luo, W., & Zhang, K. (2023). New Trends in Machine Translation using Large Language Models: Case Examples with ChatGPT. *International Joint Conference on Artificial Intelligence (IJCAI)*. Lyu, C., Liu, S., Wu, M., Wang, L., Luo, W., & Zhang, K. (2023). New Trends in Machine Translation using Large Language Models: Case Examples with ChatGPT. *arXiv preprint arXiv:2305.01181v2*. Wang, L., Liu, S., Wu, M., Luo, W., & Zhang, K. Recent Advances in Interactive Machine Translation with Large Language Models. *ResearchGate*. DOI: 10.13140/RG.2.2.17363.17445