BiasEdit: A Promising Approach to Debiasing AI Models
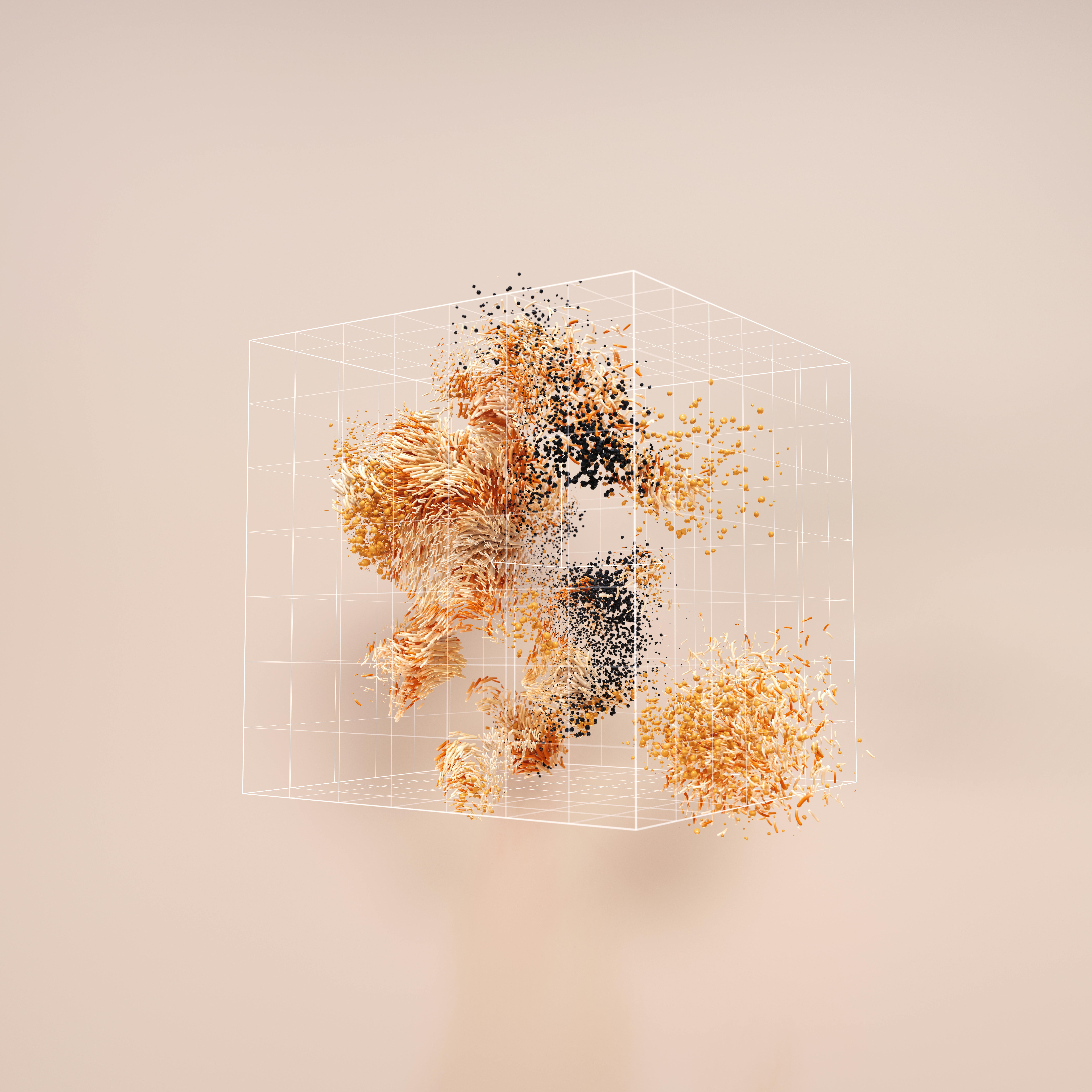
Top post
AI Models Unlearn Stereotypes: BiasEdit as a Promising Approach
Language models, the foundation of many modern AI applications, have developed rapidly in recent years. Their ability to generate human-like text opens up countless possibilities, from automated text creation to intelligent chatbots. However, these advancements also bring challenges. A central problem is the tendency of AI models to reproduce stereotypes and biases from the training data. This can lead to discriminatory or unfair outcomes and jeopardize the integrity of AI systems.
Traditional methods for combating bias in AI models, such as retraining with corrected data or adjusting the representations, often reach their limits. They are either computationally intensive, do not effectively remove stereotypes, or impair the overall performance of the model. A new approach, gaining increasing importance in the research community, is called "Model Editing".
BiasEdit, a recently introduced method, pursues precisely this approach. Instead of retraining the entire model, BiasEdit focuses on targeted adjustments of specific model parameters. This is done using small, specialized neural networks called "editors." These editors learn how to modify the parameters of the language model in such a way that stereotypical outputs are reduced without affecting the general language capabilities.
The key to BiasEdit's success lies in the combination of two loss functions: a "debiasing loss" function, which measures the reduction of stereotypes, and a "retention loss" function, which ensures that the basic language capabilities of the model are preserved. Through this balanced relationship between debiasing and maintaining model performance, BiasEdit offers an efficient and robust way to mitigate stereotypes.
Initial experiments with established datasets like StereoSet and Crows-Pairs show promising results. Compared to conventional debiasing methods, BiasEdit was able to significantly reduce stereotypes in the generated texts without significantly affecting the overall language quality of the model. Furthermore, BiasEdit enables a detailed analysis of the bias distribution within the model, which contributes to a deeper understanding of the emergence and persistence of stereotypes in AI systems.
Research in the field of model editing and debiasing is still in its early stages, but BiasEdit represents an important step towards fair and responsible AI. The targeted adjustment of model parameters offers an efficient and effective way to combat stereotypes and strengthen trust in AI systems. Future research will focus on extending the applicability of BiasEdit to more complex models and datasets and investigating the long-term stability of the debiasing effects.
Bibliography: Xu, X., Xu, W., Zhang, N., & McAuley, J. (2025). BiasEdit: Debiasing Stereotyped Language Models via Model Editing. *arXiv preprint arXiv:2503.08588*. Zhao, J., Wallace, E., Feng, S., Klein, D., & Singh, S. (2024). Calibrating Trust in Large Language Models. *arXiv preprint arXiv:2402.13462*. Burns, C., Ye, H., Klein, D., & Steinhardt, J. (2023). Discovering Language Model Behaviors with Model-Written Evaluations. *arXiv preprint arXiv:2212.09251*. Mitchell, T., Lee, B., Khaki, M., & Manning, C. D. (2024). Can We Debias Multimodal Large Language Models via Model Editing? *arXiv preprint arXiv:2502.11559*. Pryzant, R., et al. (2023). Measuring and mitigating unintended bias in text embeddings. *Proceedings of the 2023 Conference on Empirical Methods in Natural Language Processing*, 12284–12303.