AI Video Generation Advances with Long Context Tuning for Coherent Scenes
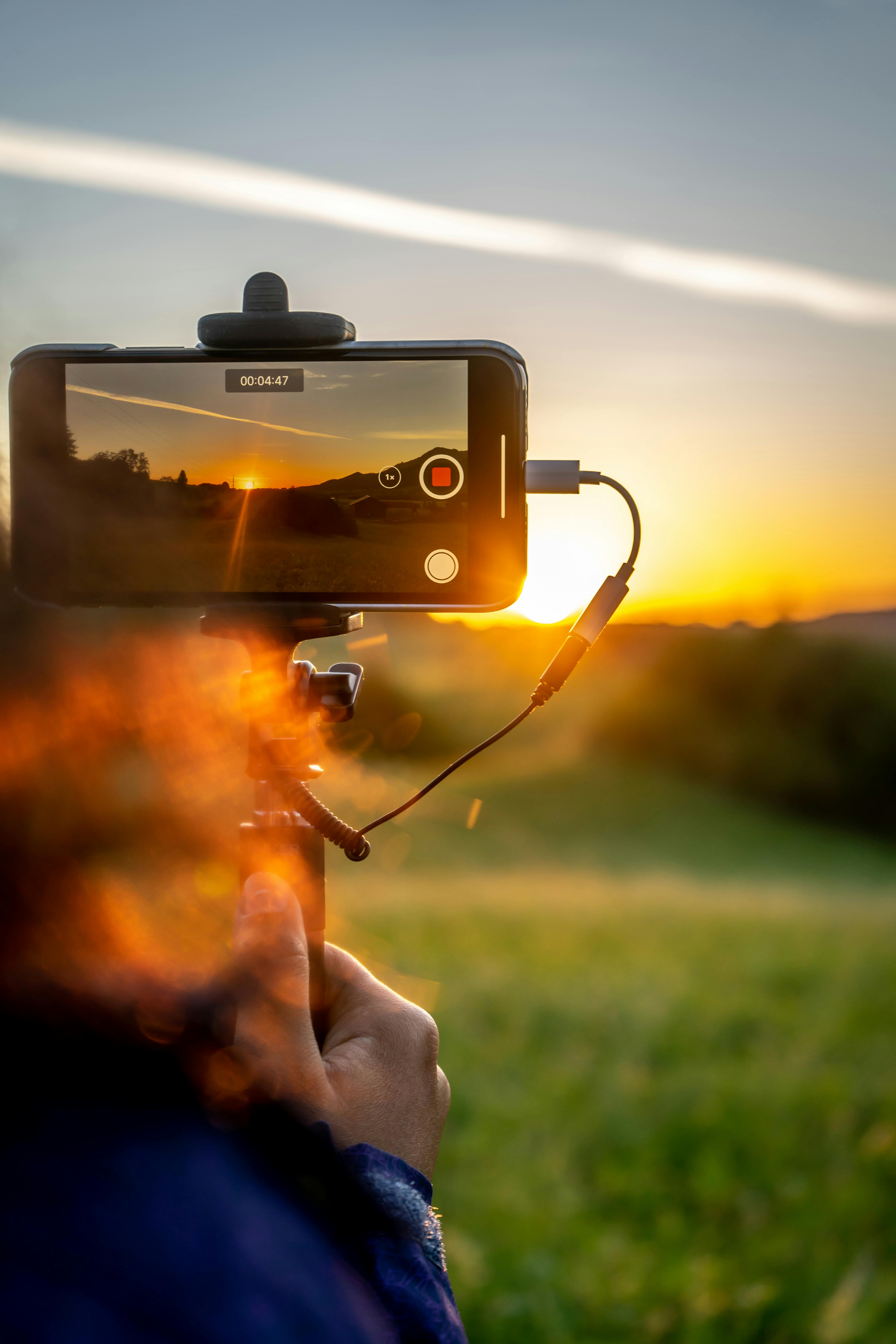
Top post
AI-Powered Video Generation Reaches New Dimensions: Multiple Coherent Scenes Thanks to "Long Context Tuning"
The generation of videos using Artificial Intelligence (AI) has made remarkable progress in recent years. Realistic, minutes-long videos in a single pass are now a reality thanks to scalable diffusion transformers. However, the demands of practical applications, especially in the field of narrative videos, go beyond such single shots. Multi-part scenes are required, which are both visually and dynamically consistent across individual sections. A new approach called "Long Context Tuning" (LCT) promises a solution.
LCT Extends the Context of AI Models
LCT is a training paradigm that extends the context window of pre-trained diffusion models for single shots. The goal is to enable the model to learn scene-wide consistency directly from the data. Instead of limiting itself to individual shots, the attention mechanisms are extended to all shots within a scene. This is achieved through the integration of interleaved 3D position embeddings and an asynchronous noise strategy. This enables both joint and autoregressive generation of shots without requiring additional parameters.
Bidirectional and Context-Causal Attention
Models with bidirectional attention after LCT training can be further optimized by fine-tuning with context-causal attention. This facilitates autoregressive generation using an efficient KV-cache (Key-Value-Cache). This cache stores the calculations of the attention layers, avoiding redundant calculations and increasing the speed of generation.
Promising Results and New Possibilities
Initial experiments show that single-shot models after LCT training are able to generate coherent scenes with multiple shots. Furthermore, promising new capabilities are emerging, including compositional generation and interactive extension of shots. These advances open new avenues for the practical creation of visual content and could revolutionize video production.
Outlook
Developments in the field of AI-powered video generation are rapid. LCT represents an important step in pushing the boundaries of what is possible and enabling the creation of more complex and coherent videos. The ability to ensure scene-wide consistency opens up new possibilities for the creative design and production of videos. Future research will focus on further improving the efficiency and scalability of LCT and exploring its application possibilities in various fields.
Bibliography: Guo, Y., Yang, C., Yang, Z., Ma, Z., Lin, Z., Yang, Z., Lin, D., & Jiang, L. (2025). Long Context Tuning for Video Generation. *arXiv preprint arXiv:2503.10589*. https://huggingface.co/papers/2503.10589 https://arxiv.org/abs/2503.08605 https://arxiv.org/abs/2501.05484 https://huggingface.co/papers https://proceedings.neurips.cc/paper_files/paper/2024/file/329ad516cf7a6ac306f29882e9c77558-Paper-Datasets_and_Benchmarks_Track.pdf https://www.reddit.com/r/ninjasaid13/comments/1jatl5v/250310589_long_context_tuning_for_video_generation/ https://openreview.net/forum?id=ijoqFqSC7p https://aclanthology.org/2024.acl-long.52/ https://github.com/yunlong10/Awesome-LLMs-for-Video-Understanding https://heidloff.net/article/long-lora/