AI-Powered Health Assistants on Edge Devices: A New Approach to Personalized Medicine
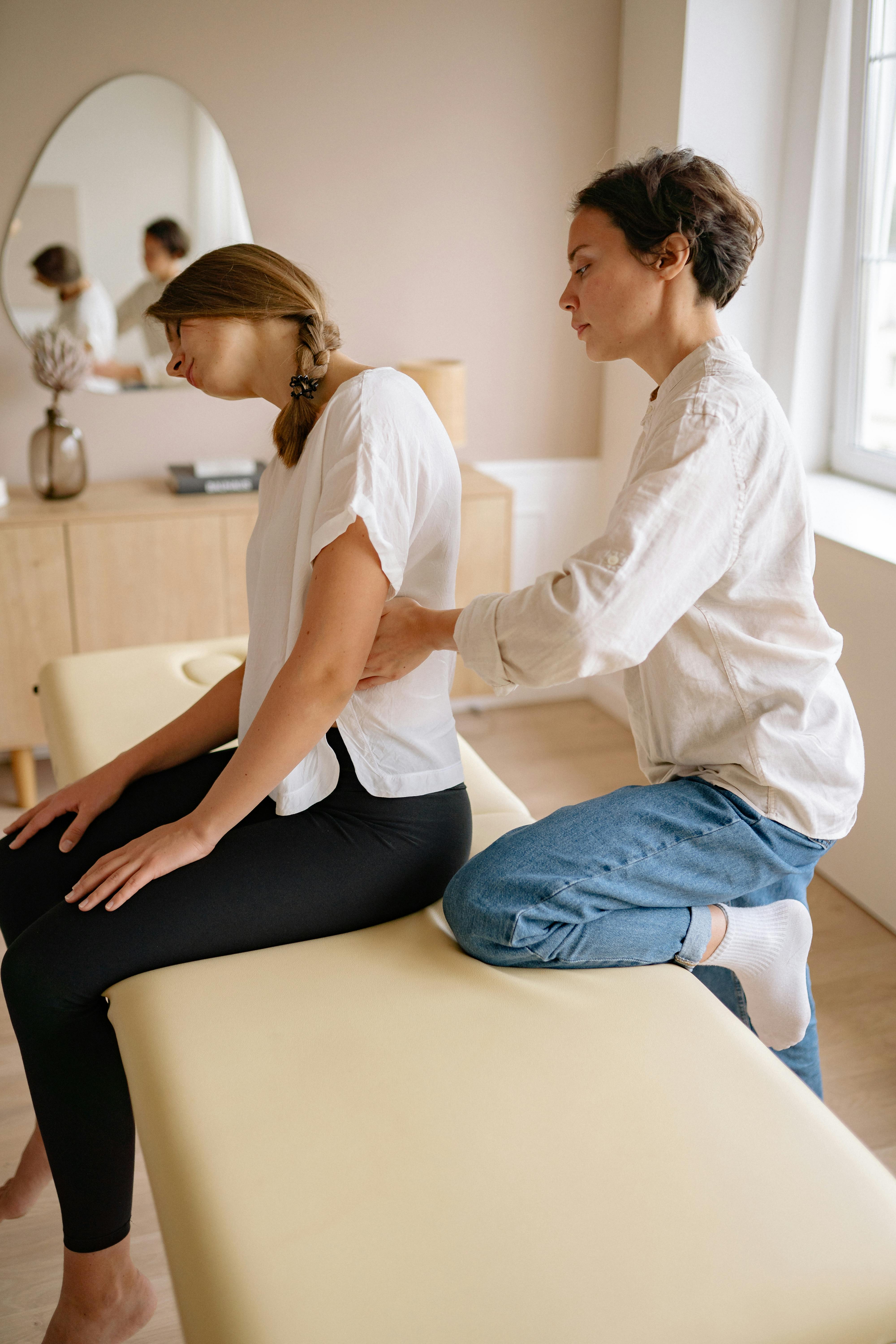
Top post
AI-Powered Health Assistants on Edge Devices: A New Approach to Personalized Medicine
The rapid development of Artificial Intelligence (AI) opens up promising possibilities in healthcare. In particular, so-called Large Action Models (LAMs) have the potential to drive intelligent automation in this area. However, several hurdles currently stand in the way of their widespread use in healthcare. Data privacy concerns, latency issues, and the dependence on a stable internet connection pose significant challenges.
A promising solution to overcome these challenges is AI-based health assistants that run directly on edge devices, such as smartphones or smartwatches. This approach enables decentralized data processing, which protects sensitive patient data and minimizes latency. Furthermore, the dependence on a permanent internet connection is reduced, which increases the availability and reliability of the application.
Multi-Agent Systems for Efficient Resource Utilization
An innovative approach in this area relies on so-called multi-agent systems. Multiple specialized AI agents work together to perform different tasks. For example, one agent could be responsible for scheduling appointments, while another agent monitors the user's health status and sends medication reminders. This division of labor allows for more efficient use of resources and improves the scalability of the system.
An example of such a system is a recently introduced multi-agent-based health assistant for edge devices. This assistant offers various functions, including appointment booking, health monitoring, medication reminders, and daily health reports. The individual agents are trained for specific tasks and communicate with each other to ensure a seamless user experience.
High-Performance AI Models for Use on Edge Devices
The development of powerful, yet resource-efficient AI models is crucial for the success of AI assistants on edge devices. Models like Qwen Code Instruct 2.5 7B demonstrate that it is possible to achieve high accuracy with low resource requirements. This allows the execution of complex AI algorithms directly on the device without negatively impacting performance or battery life. The achieved results, such as a RougeL score of 85.5 for planning and 96.5 for calls, underscore the potential of this technology.
Future Perspectives and Challenges
AI-powered health assistants on edge devices have the potential to revolutionize personalized medicine. By combining powerful AI models and multi-agent architectures, individual needs and preferences of users can be taken into account. Decentralized data processing and independence from a permanent internet connection also offer advantages in terms of data privacy and reliability.
Despite the promising outlook, there are also challenges that need to be addressed. The development of robust and secure AI systems that meet the high demands of the healthcare sector is an ongoing process. User acceptance and trust in this technology also play a crucial role in its success.
Bibliography: https://arxiv.org/abs/2503.05397 https://arxiv.org/html/2503.05397v1 https://www.researchgate.net/publication/383930712_Edge_Computing_in_Healthcare_Innovations_Opportunities_and_Challenges https://www.researchgate.net/publication/318152416_Supporting_Health_and_Community_Care_with_Multi-Agent_Systems https://www.sciencedirect.com/science/article/pii/S2405959522000753 https://www.springerprofessional.de/developing-a-multi-agent-based-modeling-for-smart-search-and-res/16446976 https://github.com/AGI-Edgerunners/LLM-Agents-Papers https://www.mdpi.com/1424-8220/22/8/2820 https://pmc.ncbi.nlm.nih.gov/articles/PMC10519219/ https://www.connectedpapers.com/main/73b24c17e59a7c0102d66d4fffe4ba773157f3f6