An Empirical Study of GPT-4o's Image Generation Capabilities
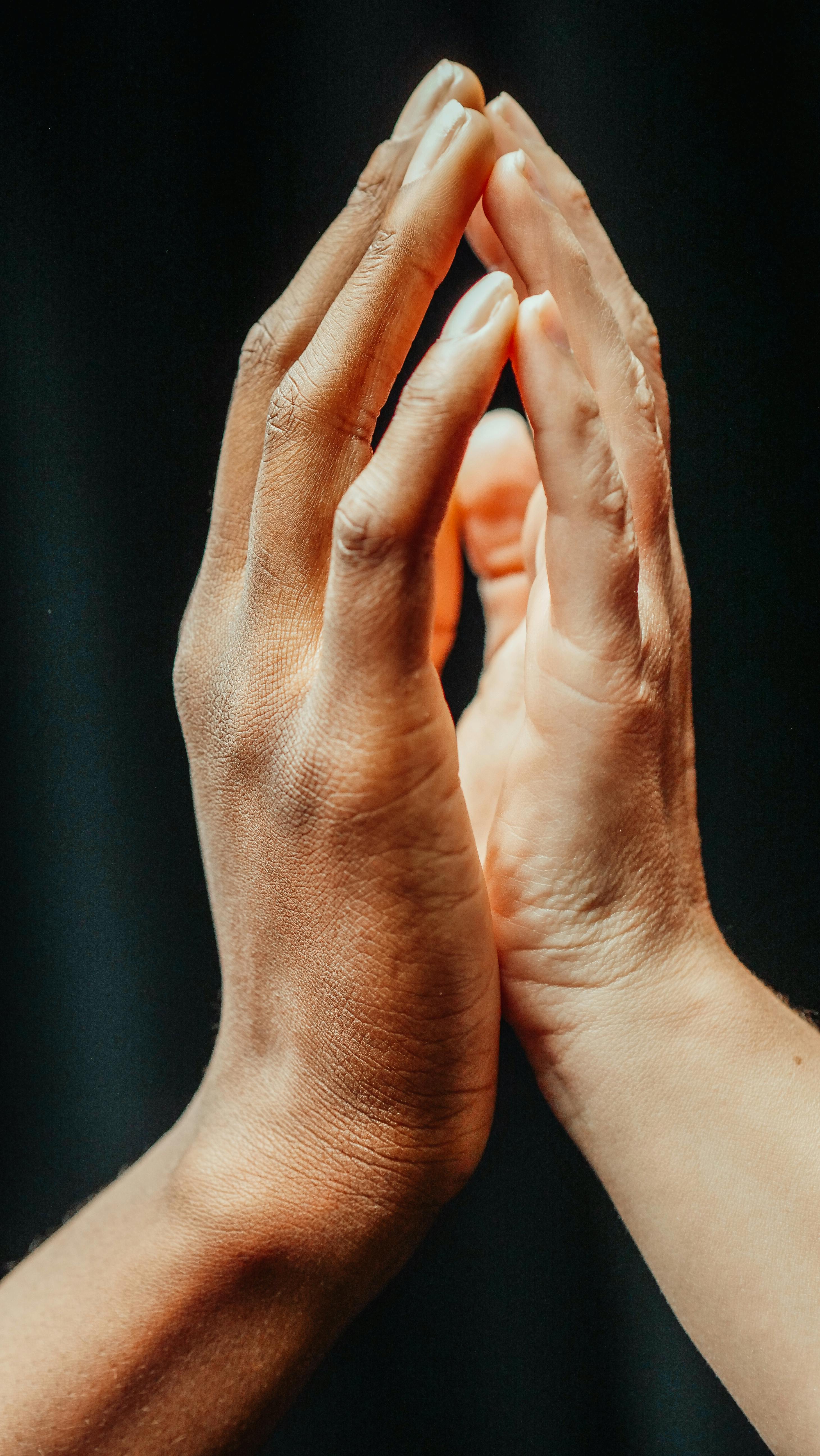
Top post
GPT-4o: An Empirical Study of its Image Generation Capabilities
Image generation has undergone rapid development in recent years. From early GAN-based approaches to diffusion models and unified generative architectures that aim to combine understanding and generation tasks, the technology has continuously evolved. In particular, models like GPT-4o have demonstrated the feasibility of multimodal generation with high fidelity. However, since the architecture of these models often remains secret and unpublished, the question arises whether image and text generation have already been successfully integrated into a unified framework in these methods.
A recent empirical study investigates the image generation capabilities of GPT-4o and compares them with leading open-source and commercial models. The evaluation encompassed four main categories: text-to-image, image-to-image, image-to-3D, and image-to-X generation, with a total of over 20 tasks. The analysis highlights the strengths and weaknesses of GPT-4o under various conditions and positions the model within the broader development of generative models.
Strengths and Weaknesses of GPT-4o
The study shows that GPT-4o possesses an impressive ability to link visual and linguistic information. In many of the tasks examined, including text-to-image, image-to-image, and image-to-3D generation, GPT-4o achieved results comparable to those of other leading models. This suggests that the integration of image and text generation within a unified framework is already well advanced.
Despite the promising results, the study also reveals some limitations of GPT-4o. Inconsistencies in generation, hallucinations, and biases in the generated images were observed. In particular, weaknesses were apparent in the representation of underrepresented cultural elements and non-Latin scripts. These observations underscore the current challenges in the design and training of such models and the importance of a comprehensive and diverse database.
The Importance of Architecture, Data, and Training
The study emphasizes that architecture alone does not determine the success of a generative model. The quality and size of the training data, as well as the optimization strategies used, play an equally important role. GPT-4o benefits from an extensive dataset and advanced training methods, which contribute to its impressive performance. At the same time, the observed weaknesses highlight the need for further research and development to improve the robustness and reliability of generative models.
Outlook on Future Developments
The investigation of GPT-4o's capabilities provides valuable insights into the current state of unified generative modeling. It identifies promising directions for future research and development, particularly with regard to architecture design, data scaling, and optimization strategies. A deeper understanding of proprietary systems like GPT-4o is crucial to advance progress in this field and promote the development of robust, fair, and creative generative models.
Research on multimodal models like GPT-4o is dynamic and promising. Further empirical studies are necessary to better understand the complex relationships between architecture, data, and training and to enable the development of innovative applications in various fields. The integration of image and text understanding and generation within a unified framework opens up diverse possibilities for the future of artificial intelligence.
Bibliography: - https://arxiv.org/abs/2504.02782 - https://openai.com/index/introducing-4o-image-generation/ - https://paperflix.es/pdfs/2025-04-06/2504.02782.pdf - https://huggingface.co/papers/2504.02782 - http://arxiv.org/pdf/2412.10587 - https://www.heise.de/en/background/Image-generator-from-GPT-4o-what-is-probably-behind-the-technical-breakthrough-10343544.html - https://dl.acm.org/doi/10.1145/3660767 - https://cdn.openai.com/papers/gpt-4.pdf - https://www.researchgate.net/publication/381308321_Unveiling_the_Safety_of_GPT-4o_An_Empirical_Study_using_Jailbreak_Attacks - https://huggingface.co/papers?q=gpt-4