Missing Premises Exacerbate Overthinking in AI Models
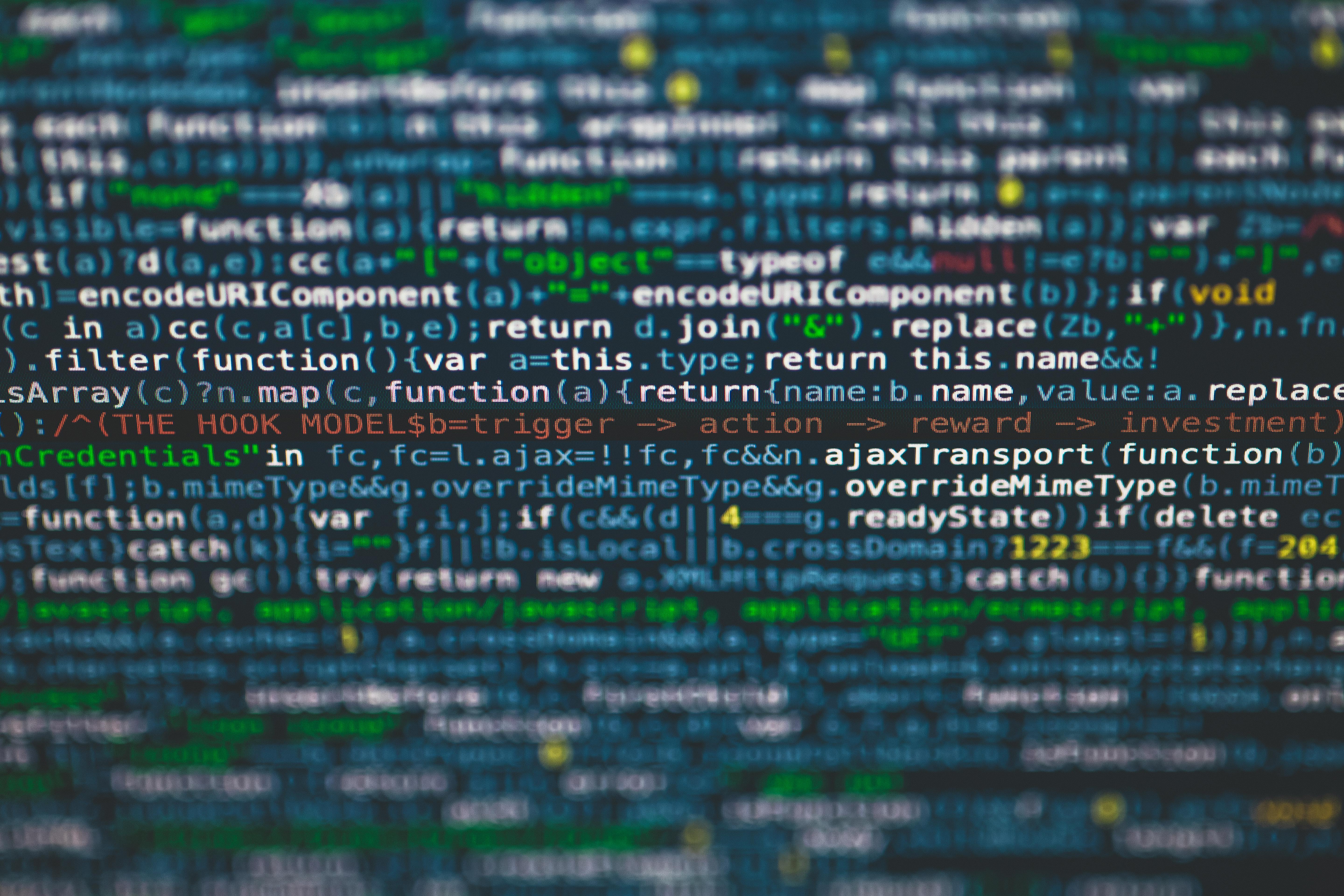
Top post
Missing Premises Amplify "Overthinking" in AI Models
Artificial intelligence (AI) has made rapid progress in recent years, particularly in the field of machine learning and natural language processing. Large language models (LLMs) are capable of handling complex tasks such as text generation, translation, and answering questions. However, recent research highlights a critical problem with these models: so-called "overthinking," an excessive and inefficient thought process, especially with questions containing missing premises.
The study shows that the response length of LLMs, regardless of whether they were trained through reinforcement learning or supervised learning, increases drastically when questions are incomplete and lack crucial information. This phenomenon, referred to as "MiP-Overthinking" (Missing Premise-Overthinking), exacerbates the general problem of overthinking and leads to redundant and ineffective thought processes. The models get lost in unnecessary details and produce long, rambling answers instead of identifying the missing information and marking the question as incomplete.
Interestingly, LLMs not specifically trained for logical reasoning perform significantly better in this scenario. They provide shorter answers and recognize the incomplete questions more quickly. This points to a critical flaw in the current training process of specialized reasoning LLMs. The focus on complex thought processes seems to impair the ability for efficient and critical thinking. The models tend to over-apply learned thinking patterns instead of questioning the validity of the input data.
To investigate the causes of this behavior more closely, the researchers analyzed the length of the responses, the overthinking patterns, and the point at which critical thinking is employed in different types of LLMs. The results show that overthinking can even be "contagious" through the distillation of answers from other reasoning models. A model trained with the responses of an overthinking model adopts this behavior and potentially amplifies it.
These findings are relevant for the development and deployment of AI systems, especially in the context of companies like Mindverse, which specialize in the development of customized AI solutions. Understanding and mitigating overthinking is crucial for creating robust and reliable AI applications, such as chatbots, voicebots, AI search engines, and knowledge systems. Only when AI models are capable of efficient and critical thinking can they reach their full potential and offer real added value to users.
The research results underscore the need to develop new training methods that promote critical thinking and effectively address the overthinking problem. Future AI systems should be able to identify missing information and react accordingly, instead of getting lost in unproductive thought processes. This is an important step towards the responsible and sustainable development of artificial intelligence.
Bibliography:
- https://chatpaper.com/chatpaper/?id=2&date=1744214400&page=1
- https://www.anthropic.com/research/reasoning-models-dont-say-think
- https://www.reddit.com/r/ClaudeAI/comments/1jr1s26/anthropic_research_paper_reasoning_models_dont/
- https://slejournal.springeropen.com/articles/10.1186/s40561-024-00316-7
- https://arxiv.org/pdf/2502.08235
- https://x.com/DachengLi177/status/1890918752423952814
- https://news.ycombinator.com/item?id=42852866
- https://www.paperdigest.org/2020/04/recent-papers-on-question-answering/
- https://assets.anthropic.com/m/71876fabef0f0ed4/original/reasoning_models_paper.pdf
- https://www.boeckler.de/fpdf/HBS-008498/p_hsi_wp_17.pdf