Decoupled Diffusion Transformers Improve Image Generation Speed and Quality
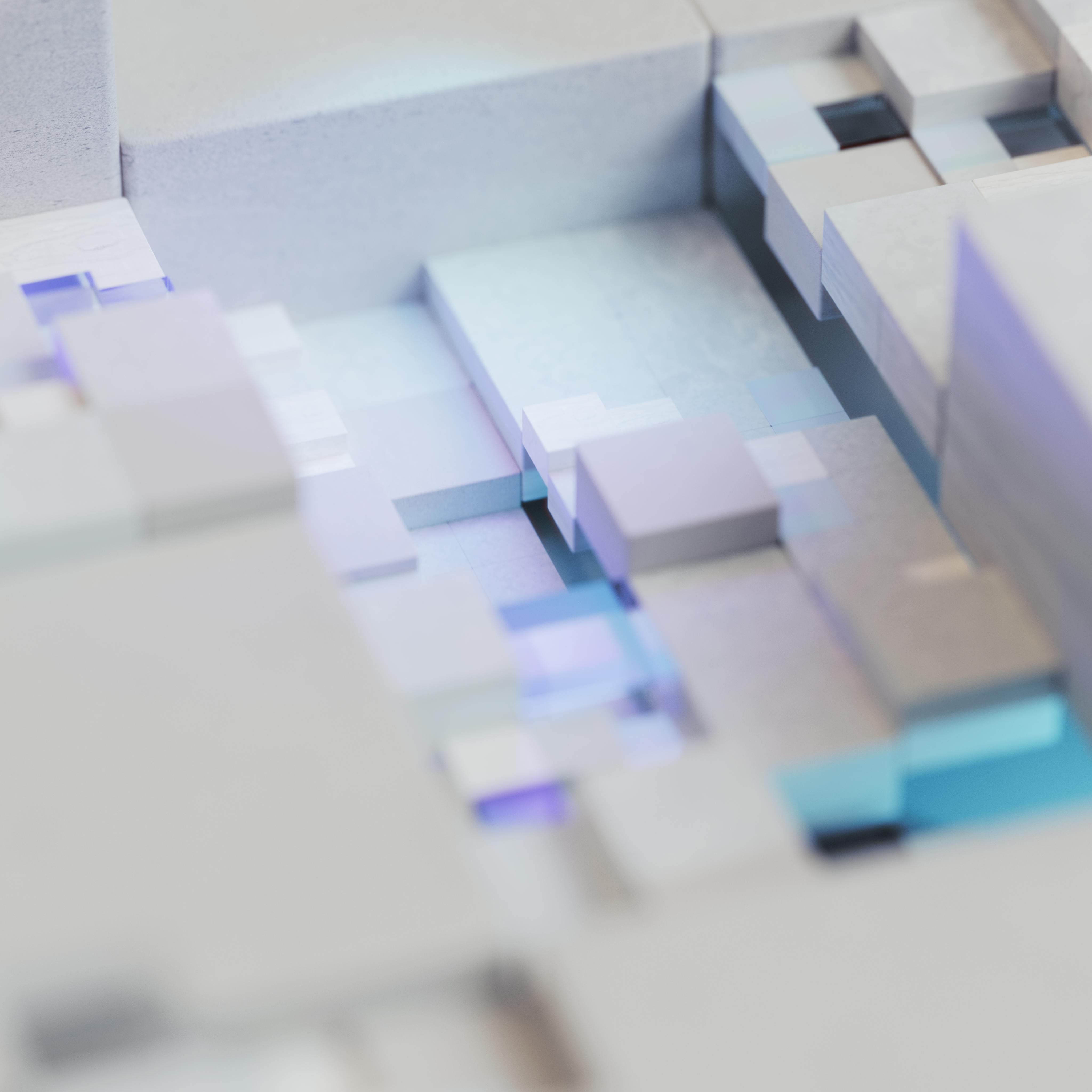
Top post
Decoupled Diffusion Transformers: A New Approach for Image Generation
Diffusion transformers have shown promise for generating high-quality images. However, they often require long training times and numerous inference steps. In each denoising step, these models encode noisy inputs to extract low-frequency semantic components and then decode the higher-frequency details with identical modules. This approach leads to an optimization dilemma: encoding low-frequency semantics requires the reduction of high-frequency components, creating a conflict between semantic encoding and high-frequency decoding.
To address this problem, the Decoupled Diffusion Transformer (DDT) has been developed. It features a decoupled design that uses a dedicated condition encoder for semantic extraction and a specialized velocity decoder. This separation allows for more efficient processing of the different frequency ranges and thus optimizes the training process.
Architecture and Advantages of DDT
Unlike conventional diffusion transformers, which use identical modules for encoding and decoding, the DDT separates these two processes. The condition encoder focuses on extracting semantic information from the noisy image, while the velocity decoder reconstructs the high-frequency details. Experiments have shown that a more powerful encoder improves performance with increasing model size.
The DDT offers several advantages over conventional diffusion transformers:
First, the decoupled architecture enables faster convergence during training. Tests with DDT-XL/2 on ImageNet 256x256 showed almost four times faster convergence compared to previous diffusion transformers and achieved a new state-of-the-art FID score of 1.31. On ImageNet 512x512, DDT-XL/2 achieved an FID of 1.28.
Second, the architecture improves inference speed by enabling the sharing of self-conditioning between adjacent denoising steps. To minimize performance degradation, a statistical dynamic programming approach was developed to identify optimal sharing strategies.
Outlook and Significance
The DDT represents a significant advancement in the field of image generation with diffusion transformers. The decoupled architecture allows for more efficient use of computational resources and leads to faster convergence and improved inference speed. Future research could focus on further optimizing the architecture and applying the DDT to other areas of generative AI.