AI Achieves Milestone in Understanding Soccer Videos
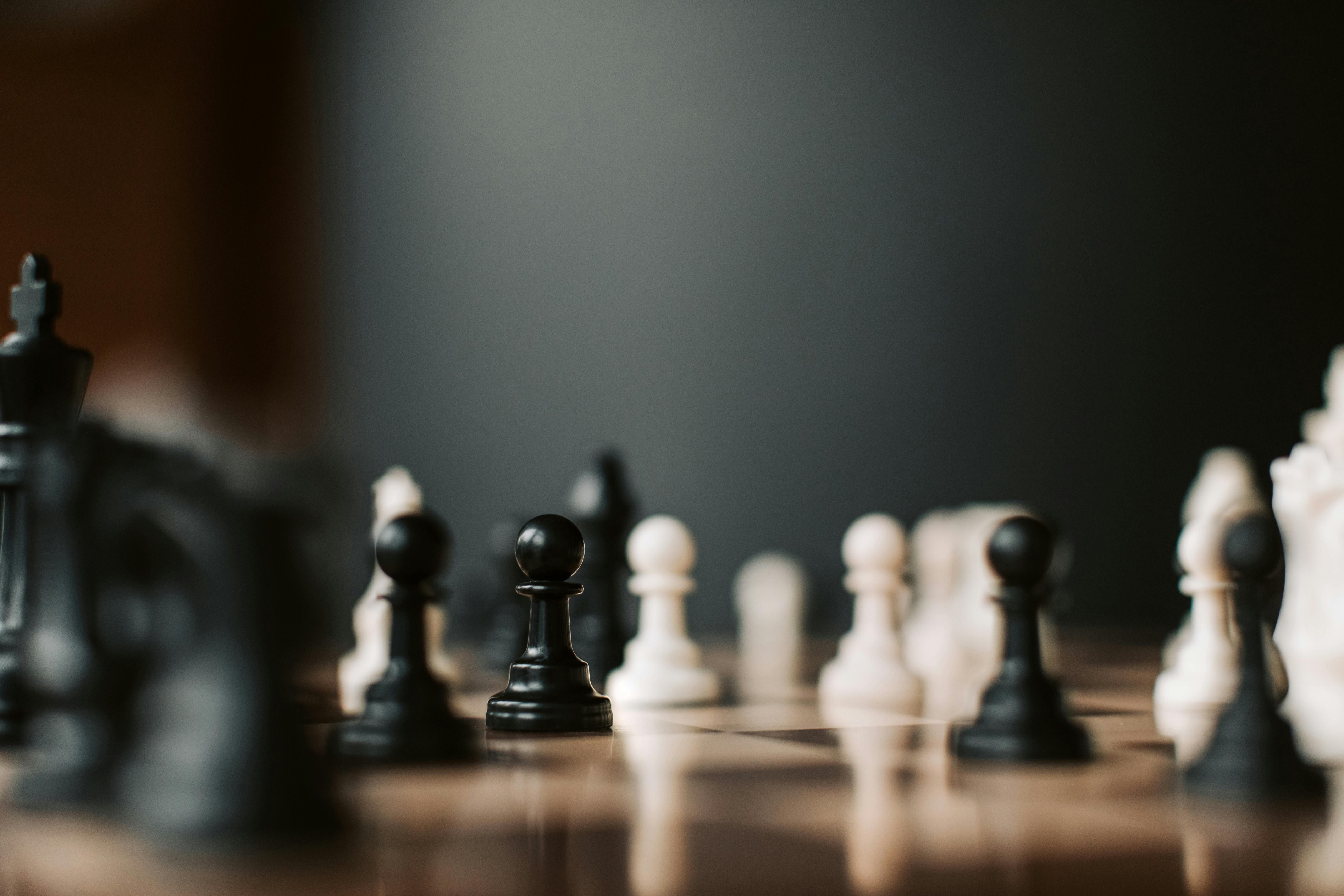
Top post
A Milestone in AI Understanding of Soccer Videos
Soccer captivates billions of fans worldwide. The complexity of the game and the ever-growing demand for detailed analysis and an enhanced viewing experience have greatly fueled research interest in applying Artificial Intelligence (AI) to interpret soccer videos. AI systems enable, for example, tactical analysis, automated content creation, and improved fan interaction.
Previous Approaches and their Limitations
Previous research in soccer video analysis has mainly focused on specialized models tailored to narrowly defined tasks. This led to limited compatibility between the models. One example is the SoccerNet datasets, which, while encompassing 500 full soccer matches, have primarily been used to develop models for specific tasks like event classification or commentary generation. A unified analytical framework that seamlessly integrates the diverse requirements of soccer video interpretation has been lacking until now.
SoccerReplay-1988: A New, Comprehensive Dataset
To create a solid foundation for understanding soccer, the largest soccer dataset to date, SoccerReplay-1988, has been created. This dataset includes 1,988 full soccer matches from six top European leagues and championships from the 2014/15 to 2023/24 seasons. For each match, the dataset contains textual commentary with second-precise timestamps as well as detailed metadata about the games, players, coaches, referees, and teams. Part of the commentary is annotated with specific event types such as corner kicks or goals. The dataset is divided into training, validation, and test data to enable the development and evaluation of AI models.
MatchVision: A Universal AI Model for Soccer
Based on SoccerReplay-1988, MatchVision has been developed, the first visual language model specifically tailored to various soccer tasks. MatchVision utilizes state-of-the-art visual language models as a foundation and enhances the image information through temporal attention. By training with diverse visual and linguistic tasks on SoccerReplay-1988, MatchVision demonstrates high adaptability to various tasks such as event classification and commentary generation.
The Strengths of MatchVision
MatchVision utilizes spatio-temporal information from soccer videos, enabling a comprehensive understanding of the game's events. It can classify events, generate commentary, and detect fouls from various perspectives. Extensive experiments and ablation studies have demonstrated MatchVision's superiority over existing models in various tasks.
Outlook and Significance for the Future
SoccerReplay-1988 and MatchVision offer a new paradigm for sports video analysis. The combination of a comprehensive dataset and a universal AI model enables the development of robust and comprehensive solutions for understanding soccer. The research results can drive the development of new applications in sports analysis, automated content creation, and fan interaction. Future research could focus on expanding the dataset to include more leagues and competitions, as well as integrating further data modalities such as audio. The investigation of ethical aspects related to the use of AI systems in sports analysis is also important.
Bibliography Rao, J., Wu, H., Jiang, H., Zhang, Y., Wang, Y., & Xie, W. (2024). Towards Universal Soccer Video Understanding. arXiv preprint arXiv:2412.01820. Rao, J., Wu, H., Jiang, H., Zhang, Y., Wang, Y., & Xie, W. (2024). Towards Universal Soccer Video Understanding. arXiv preprint arXiv:2412.01820v1. Rao, J. UniSoccer. https://jyrao.github.io/UniSoccer/ Rao, J. UniSoccer. https://github.com/jyrao/UniSoccer Giancola, S., Cioppa, A., Ghanem, B., & Van Droogenbroeck, M. (2019). Comprehensive Soccer Video Understanding: Towards Human-comparable Video Understanding System in Constrained Environment. In Proceedings of the IEEE/CVF International Conference on Computer Vision Workshops (pp. 0-0). Wu, H. Towards Universal Soccer Video Understanding. https://www.chatpaper.com/chatpaper/?id=4&date=1733155200&page=1 Xu, S., Zhu, Y., Li, G., & Wang, C. (2024). Deep Understanding of Soccer Match Videos. arXiv preprint arXiv:2407.08200. CVsports. https://openaccess.thecvf.com/CVPR2024_workshops/CVsports Karpathy, A., & Fei-Fei, L. (2017). What I learned from cs231n. https://cs231n.stanford.edu/reports/2017/pdfs/717.pdf