Alias-Free Latent Diffusion Models Enhance Consistency in Image Generation
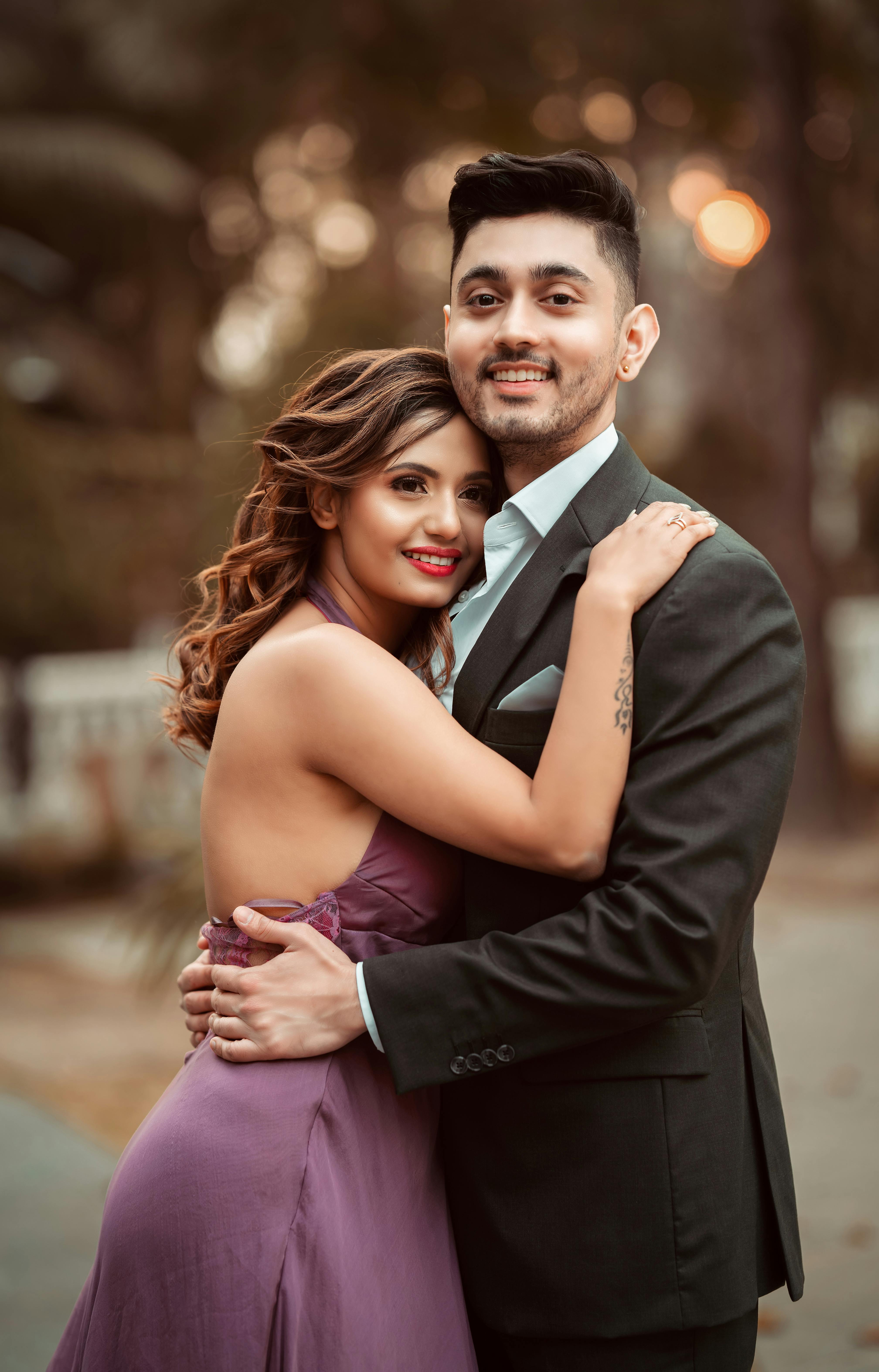
Top post
Alias-Free Latent Diffusion Models: Improved Consistency in Image Generation
Latent Diffusion Models (LDMs) have established themselves as powerful tools for image synthesis. They enable the generation of images with high resolution and detail fidelity. However, a known problem with LDMs is their instability to small changes in input. Even small shifts or perturbations in the input noise can lead to significantly different results. This inconsistency limits the applicability of LDMs in areas that require reproducible results, such as video editing or image-to-image translation.
A research team has addressed this problem and developed a method to improve the consistency of LDMs. Their approach aims to minimize so-called aliasing effects and increase the shift equivariance of the models. Shift equivariance means that a shift in the input leads to a corresponding shift in the output, without otherwise changing the generated image.
The instability of LDMs can be attributed to several factors. Firstly, aliasing effects can be amplified during the training of the variational autoencoder (VAE) and the multiple applications of the U-Net network. Secondly, the self-attention modules used in LDMs inherently lack shift equivariance.
To overcome these challenges, the researchers propose a redesign of the LDM architecture. They modify the attention modules to make them shift-equivariant. Additionally, they introduce an equivariance loss function that effectively suppresses the frequency bandwidth of the features in the continuous domain. The result is an alias-free LDM (AF-LDM) that exhibits significantly improved shift equivariance and is robust to irregular distortions.
Improved Performance in Various Applications
The effectiveness of the new approach has been demonstrated in extensive experiments. AF-LDM achieved significantly more consistent results than conventional LDMs in various applications, including video editing, frame interpolation, super-resolution, and normal estimation. The improved stability with respect to shifts proved particularly advantageous in video editing. AF-LDM also led to higher quality results in image-to-image translation.
The research results underscore the potential of alias-free LDMs for applications requiring high consistency and robustness. The improved shift equivariance allows for more precise control over the generation process and opens up new possibilities for creative applications of LDMs. The researchers have made their code publicly available to encourage further development and application of alias-free LDMs.
The development of alias-free LDMs represents an important step towards improving the stability and consistency of generative models. Future research could focus on improving training efficiency and extending applicability to further application areas.
Bibliography: - Zhou, Yifan, et al. "Alias-Free Latent Diffusion Models: Improving Fractional Shift Equivariance of Diffusion Latent Space." arXiv preprint arXiv:2503.09419 (2025). - Rombach, Robin, et al. "High-resolution image synthesis with latent diffusion models." Proceedings of the IEEE/CVF Conference on Computer Vision and Pattern Recognition. 2022. - Xu, Zhifeng, et al. "Alias-free generative adversarial networks." Proceedings of the 39th International Conference on Machine Learning. PMLR, 2022.